技术背景
损失函数是机器学习中直接决定训练结果好坏的一个模块,该函数用于定义计算出来的结果或者是神经网络给出的推测结论与正确结果的偏差程度,偏差的越多,就表明对应的参数越差。而损失函数的另一个重要性在于会影响到优化函数的收敛性,如果损失函数的指数定义的太高,稍有参数波动就导致结果的巨大波动的话,那么训练和优化就很难收敛。一般我们常用的损失函数是MSE(均方误差)和MAE(平均标准差)等。那么这里我们尝试在MindSpore中去自定义一些损失函数,可用于适应自己的特殊场景。
MindSpore内置的损失函数
刚才提到的MSE和MAE等常见损失函数,MindSpore中是有内置的,通过net_loss = nn.loss.MSELoss()
即可调用,再传入Model
中进行训练,具体使用方法可以参考如下拟合一个非线性函数的案例:
- # test_nonlinear.py
-
- from mindspore import context
- import numpy as np
- from mindspore import dataset as ds
- from mindspore import nn, Tensor, Model
- import time
- from mindspore.train.callback import Callback, LossMonitor
- import mindspore as ms
- ms.common.set_seed(0)
-
- def get_data(num, a=2.0, b=3.0, c=5.0):
- for _ in range(num):
- x = np.random.uniform(-1.0, 1.0)
- y = np.random.uniform(-1.0, 1.0)
- noise = np.random.normal(0, 0.03)
- z = a * x ** 2 + b * y ** 3 + c + noise
- yield np.array([[x**2], [y**3]],dtype=np.float32).reshape(1,2), np.array([z]).astype(np.float32)
-
- def create_dataset(num_data, batch_size=16, repeat_size=1):
- input_data = ds.GeneratorDataset(list(get_data(num_data)), column_names=['xy','z'])
- input_data = input_data.batch(batch_size)
- input_data = input_data.repeat(repeat_size)
- return input_data
-
- data_number = 160
- batch_number = 10
- repeat_number = 10
-
- ds_train = create_dataset(data_number, batch_size=batch_number, repeat_size=repeat_number)
-
- class LinearNet(nn.Cell):
- def __init__(self):
- super(LinearNet, self).__init__()
- self.fc = nn.Dense(2, 1, 0.02, 0.02)
-
- def construct(self, x):
- x = self.fc(x)
- return x
-
- start_time = time.time()
- net = LinearNet()
- model_params = net.trainable_params()
- print ('Param Shape is: {}'.format(len(model_params)))
- for net_param in net.trainable_params():
- print(net_param, net_param.asnumpy())
- net_loss = nn.loss.MSELoss()
-
- optim = nn.Momentum(net.trainable_params(), learning_rate=0.01, momentum=0.6)
- model = Model(net, net_loss, optim)
-
- epoch = 1
- model.train(epoch, ds_train, callbacks=[LossMonitor(10)], dataset_sink_mode=True)
-
- for net_param in net.trainable_params():
- print(net_param, net_param.asnumpy())
-
- print ('The total time cost is: {}s'.format(time.time() - start_time))
训练的结果如下:
- epoch: 1 step: 160, loss is 2.5267093
- Parameter (name=fc.weight, shape=(1, 2), dtype=Float32, requires_grad=True) [[1.0694231 0.12706374]]
- Parameter (name=fc.bias, shape=(1,), dtype=Float32, requires_grad=True) [5.186701]
- The total time cost is: 8.412306308746338s
最终优化出来的loss值是2.5,不过在损失函数定义不同的情况下,单纯只看loss值是没有意义的。所以通常是大家统一定一个测试的标准,比如大家都用MAE来衡量最终训练出来的模型的好坏,但是中间训练的过程不一定采用MAE来作为损失函数。
自定义损失函数
由于python语言的灵活性,使得我们可以继承基本类和函数,只要使用mindspore允许范围内的算子,就可以实现自定义的损失函数。我们先看一个简单的案例,暂时将我们自定义的损失函数命名为L1Loss:
- # test_nonlinear.py
-
- from mindspore import context
- import numpy as np
- from mindspore import dataset as ds
- from mindspore import nn, Tensor, Model
- import time
- from mindspore.train.callback import Callback, LossMonitor
- import mindspore as ms
- import mindspore.ops as ops
- from mindspore.nn.loss.loss import Loss
- ms.common.set_seed(0)
-
- def get_data(num, a=2.0, b=3.0, c=5.0):
- for _ in range(num):
- x = np.random.uniform(-1.0, 1.0)
- y = np.random.uniform(-1.0, 1.0)
- noise = np.random.normal(0, 0.03)
- z = a * x ** 2 + b * y ** 3 + c + noise
- yield np.array([[x**2], [y**3]],dtype=np.float32).reshape(1,2), np.array([z]).astype(np.float32)
-
- def create_dataset(num_data, batch_size=16, repeat_size=1):
- input_data = ds.GeneratorDataset(list(get_data(num_data)), column_names=['xy','z'])
- input_data = input_data.batch(batch_size)
- input_data = input_data.repeat(repeat_size)
- return input_data
-
- data_number = 160
- batch_number = 10
- repeat_number = 10
-
- ds_train = create_dataset(data_number, batch_size=batch_number, repeat_size=repeat_number)
-
- class LinearNet(nn.Cell):
- def __init__(self):
- super(LinearNet, self).__init__()
- self.fc = nn.Dense(2, 1, 0.02, 0.02)
-
- def construct(self, x):
- x = self.fc(x)
- return x
-
- start_time = time.time()
- net = LinearNet()
- model_params = net.trainable_params()
- print ('Param Shape is: {}'.format(len(model_params)))
- for net_param in net.trainable_params():
- print(net_param, net_param.asnumpy())
-
- class L1Loss(Loss):
- def __init__(self, reduction="mean"):
- super(L1Loss, self).__init__(reduction)
- self.abs = ops.Abs()
-
- def construct(self, base, target):
- x = self.abs(base - target)
- return self.get_loss(x)
-
- user_loss = L1Loss()
-
- optim = nn.Momentum(net.trainable_params(), learning_rate=0.01, momentum=0.6)
- model = Model(net, user_loss, optim)
-
- epoch = 1
- model.train(epoch, ds_train, callbacks=[LossMonitor(10)], dataset_sink_mode=True)
-
- for net_param in net.trainable_params():
- print(net_param, net_param.asnumpy())
-
- print ('The total time cost is: {}s'.format(time.time() - start_time))
这里自己定义的内容实际上有两个部分,一个是construct函数中的计算结果的函数,比如这里使用的是求绝对值。另外一个定义的部分是reduction参数,我们从mindspore的源码中可以看到,这个reduction函数可以决定调用哪一种计算方法,定义好的有平均值、求和、保持不变三种策略。
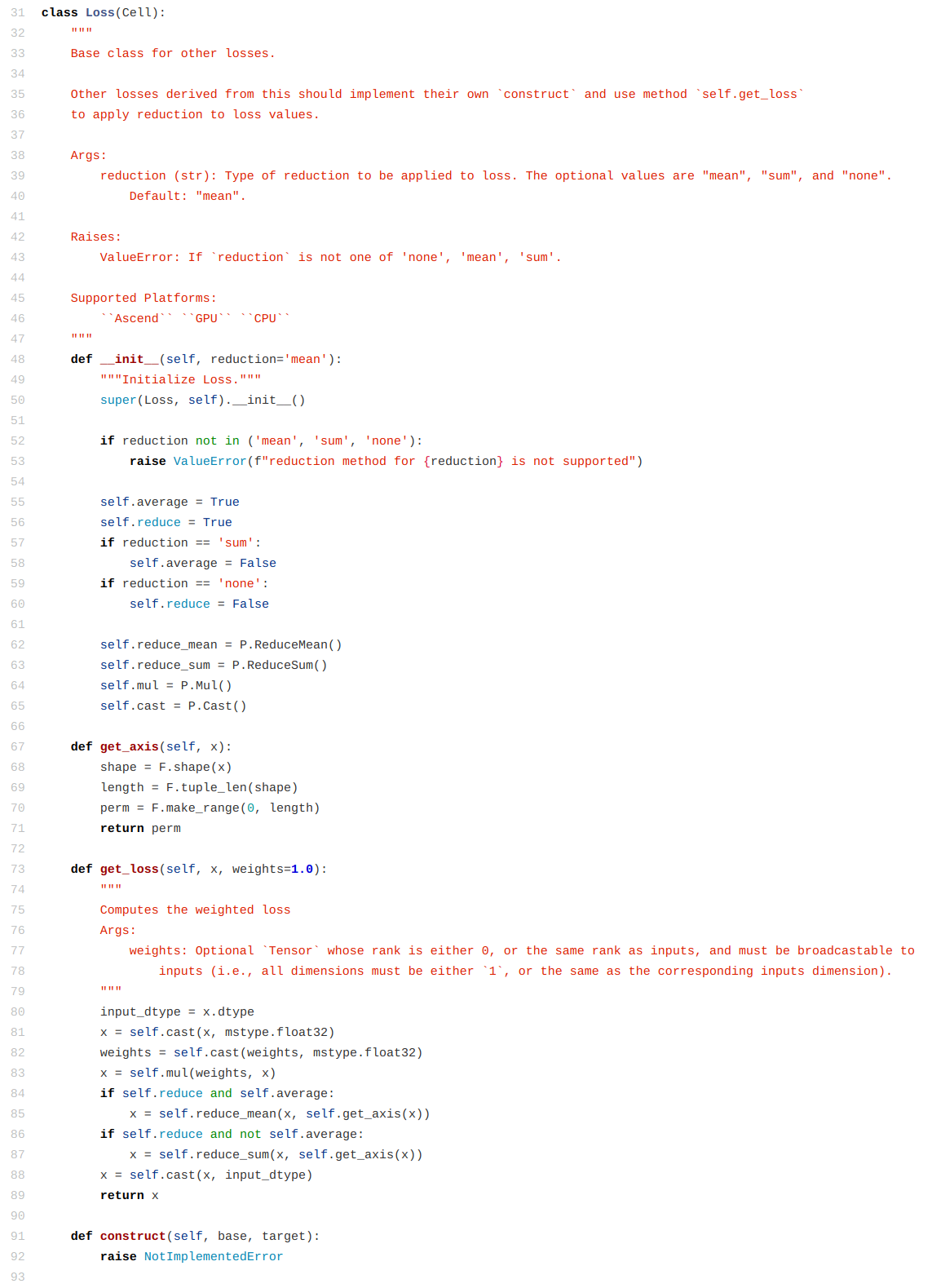
那么最后看下自定义的这个损失函数的运行结果:
- epoch: 1 step: 160, loss is 1.8300734
- Parameter (name=fc.weight, shape=(1, 2), dtype=Float32, requires_grad=True) [[ 1.2687287 -0.09565887]]
- Parameter (name=fc.bias, shape=(1,), dtype=Float32, requires_grad=True) [3.7297544]
- The total time cost is: 7.0749146938323975s
这里不必太在乎loss的值,因为前面也提到了,不同的损失函数框架下,计算出来的值就是不一样的,小一点大一点并没有太大意义,最终还是需要大家统一一个标准才能够进行很好的衡量和对比。
自定义其他算子
类似于上面一个章节的案例,这里我们仅仅是替换了一个abs的算子为square的算子,从求绝对值变化到求均方误差,这里只是修改了一个算子,内容较为简单:
- # test_nonlinear.py
-
- from mindspore import context
- import numpy as np
- from mindspore import dataset as ds
- from mindspore import nn, Tensor, Model
- import time
- from mindspore.train.callback import Callback, LossMonitor
- import mindspore as ms
- import mindspore.ops as ops
- from mindspore.nn.loss.loss import Loss
- ms.common.set_seed(0)
-
- def get_data(num, a=2.0, b=3.0, c=5.0):
- for _ in range(num):
- x = np.random.uniform(-1.0, 1.0)
- y = np.random.uniform(-1.0, 1.0)
- noise = np.random.normal(0, 0.03)
- z = a * x ** 2 + b * y ** 3 + c + noise
- yield np.array([[x**2], [y**3]],dtype=np.float32).reshape(1,2), np.array([z]).astype(np.float32)
-
- def create_dataset(num_data, batch_size=16, repeat_size=1):
- input_data = ds.GeneratorDataset(list(get_data(num_data)), column_names=['xy','z'])
- input_data = input_data.batch(batch_size)
- input_data = input_data.repeat(repeat_size)
- return input_data
-
- data_number = 160
- batch_number = 10
- repeat_number = 10
-
- ds_train = create_dataset(data_number, batch_size=batch_number, repeat_size=repeat_number)
-
- class LinearNet(nn.Cell):
- def __init__(self):
- super(LinearNet, self).__init__()
- self.fc = nn.Dense(2, 1, 0.02, 0.02)
-
- def construct(self, x):
- x = self.fc(x)
- return x
-
- start_time = time.time()
- net = LinearNet()
- model_params = net.trainable_params()
- print ('Param Shape is: {}'.format(len(model_params)))
- for net_param in net.trainable_params():
- print(net_param, net_param.asnumpy())
-
- class L1Loss(Loss):
- def __init__(self, reduction="mean"):
- super(L1Loss, self).__init__(reduction)
- self.square = ops.Square()
-
- def construct(self, base, target):
- x = self.square(base - target)
- return self.get_loss(x)
-
- user_loss = L1Loss()
-
- optim = nn.Momentum(net.trainable_params(), learning_rate=0.01, momentum=0.6)
- model = Model(net, user_loss, optim)
-
- epoch = 1
- model.train(epoch, ds_train, callbacks=[LossMonitor(10)], dataset_sink_mode=True)
-
- for net_param in net.trainable_params():
- print(net_param, net_param.asnumpy())
-
- print ('The total time cost is: {}s'.format(time.time() - start_time))
关于更多的算子内容,可以参考下这个链接(https://www.mindspore.cn/doc/api_python/zh-CN/r1.2/mindspore/mindspore.ops.html)中的内容,上述代码的运行结果如下:
- epoch: 1 step: 160, loss is 2.5267093
- Parameter (name=fc.weight, shape=(1, 2), dtype=Float32, requires_grad=True) [[1.0694231 0.12706374]]
- Parameter (name=fc.bias, shape=(1,), dtype=Float32, requires_grad=True) [5.186701]
- The total time cost is: 6.87545919418335s
可以从这个结果中发现的是,计算出来的结果跟最开始使用的内置的MSELoss结果是一样的,这是因为我们自定义的这个求损失函数的形式与内置的MSE是吻合的。
多层算子的应用
上面的两个例子都是简单的说明了一下通过单个算子构造的损失函数,其实如果是一个复杂的损失函数,也可以通过多个算子的组合操作来进行实现:
- # test_nonlinear.py
-
- from mindspore import context
- import numpy as np
- from mindspore import dataset as ds
- from mindspore import nn, Tensor, Model
- import time
- from mindspore.train.callback import Callback, LossMonitor
- import mindspore as ms
- import mindspore.ops as ops
- from mindspore.nn.loss.loss import Loss
- ms.common.set_seed(0)
-
- def get_data(num, a=2.0, b=3.0, c=5.0):
- for _ in range(num):
- x = np.random.uniform(-1.0, 1.0)
- y = np.random.uniform(-1.0, 1.0)
- noise = np.random.normal(0, 0.03)
- z = a * x ** 2 + b * y ** 3 + c + noise
- yield np.array([[x**2], [y**3]],dtype=np.float32).reshape(1,2), np.array([z]).astype(np.float32)
-
- def create_dataset(num_data, batch_size=16, repeat_size=1):
- input_data = ds.GeneratorDataset(list(get_data(num_data)), column_names=['xy','z'])
- input_data = input_data.batch(batch_size)
- input_data = input_data.repeat(repeat_size)
- return input_data
-
- data_number = 160
- batch_number = 10
- repeat_number = 10
-
- ds_train = create_dataset(data_number, batch_size=batch_number, repeat_size=repeat_number)
-
- class LinearNet(nn.Cell):
- def __init__(self):
- super(LinearNet, self).__init__()
- self.fc = nn.Dense(2, 1, 0.02, 0.02)
-
- def construct(self, x):
- x = self.fc(x)
- return x
-
- start_time = time.time()
- net = LinearNet()
- model_params = net.trainable_params()
- print ('Param Shape is: {}'.format(len(model_params)))
- for net_param in net.trainable_params():
- print(net_param, net_param.asnumpy())
-
- class L1Loss(Loss):
- def __init__(self, reduction="mean"):
- super(L1Loss, self).__init__(reduction)
- self.square = ops.Square()
-
- def construct(self, base, target):
- x = self.square(self.square(base - target))
- return self.get_loss(x)
-
- user_loss = L1Loss()
-
- optim = nn.Momentum(net.trainable_params(), learning_rate=0.01, momentum=0.6)
- model = Model(net, user_loss, optim)
-
- epoch = 1
- model.train(epoch, ds_train, callbacks=[LossMonitor(10)], dataset_sink_mode=True)
-
- for net_param in net.trainable_params():
- print(net_param, net_param.asnumpy())
-
- print ('The total time cost is: {}s'.format(time.time() - start_time))
这里使用的函数是两个平方算子,也就是四次方的均方误差,运行结果如下:
- epoch: 1 step: 160, loss is 16.992222
- Parameter (name=fc.weight, shape=(1, 2), dtype=Float32, requires_grad=True) [[0.14460069 0.32045612]]
- Parameter (name=fc.bias, shape=(1,), dtype=Float32, requires_grad=True) [5.6676607]
- The total time cost is: 7.253541946411133s
在实际的运算过程中,我们肯定不能够说提升损失函数的幂次就一定能够提升结果的优劣,但是通过多种基础算子的组合,理论上说我们在一定的误差允许范围内,是可以实现任意的一个损失函数(通过泰勒展开取截断项)的。
重定义reduction
方才提到这里面自定义损失函数的两个重点,一个是上面三个章节中所演示的construct
函数的重写,这部分实际上是重新设计损失函数的函数表达式。另一个是reduction
的自定义,这部分关系到不同的单点损失函数值之间的关系。举个例子来说,如果我们将reduction
设置为求和,那么get_loss()
这部分的函数内容就是把所有的单点函数值加起来返回一个最终的值,求平均值也是类似的。那么通过自定义一个新的get_loss()
函数,我们就可以实现更加灵活的一些操作,比如我们可以选择将所有的结果乘起来求积而不是求和(只是举个例子,大部分情况下不会这么操作)。在python中要重写这个函数也容易,就是在继承父类的自定义类中定义一个同名函数即可,但是注意我们最好是保留原函数中的一些内容,在原内容的基础上加一些东西,冒然改模块有可能导致不好定位的运行报错。
- # test_nonlinear.py
-
- from mindspore import context
- import numpy as np
- from mindspore import dataset as ds
- from mindspore import nn, Tensor, Model
- import time
- from mindspore.train.callback import Callback, LossMonitor
- import mindspore as ms
- import mindspore.ops as ops
- from mindspore.nn.loss.loss import Loss
- ms.common.set_seed(0)
-
- def get_data(num, a=2.0, b=3.0, c=5.0):
- for _ in range(num):
- x = np.random.uniform(-1.0, 1.0)
- y = np.random.uniform(-1.0, 1.0)
- noise = np.random.normal(0, 0.03)
- z = a * x ** 2 + b * y ** 3 + c + noise
- yield np.array([[x**2], [y**3]],dtype=np.float32).reshape(1,2), np.array([z]).astype(np.float32)
-
- def create_dataset(num_data, batch_size=16, repeat_size=1):
- input_data = ds.GeneratorDataset(list(get_data(num_data)), column_names=['xy','z'])
- input_data = input_data.batch(batch_size)
- input_data = input_data.repeat(repeat_size)
- return input_data
-
- data_number = 160
- batch_number = 10
- repeat_number = 10
-
- ds_train = create_dataset(data_number, batch_size=batch_number, repeat_size=repeat_number)
-
- class LinearNet(nn.Cell):
- def __init__(self):
- super(LinearNet, self).__init__()
- self.fc = nn.Dense(2, 1, 0.02, 0.02)
-
- def construct(self, x):
- x = self.fc(x)
- return x
-
- start_time = time.time()
- net = LinearNet()
- model_params = net.trainable_params()
- print ('Param Shape is: {}'.format(len(model_params)))
- for net_param in net.trainable_params():
- print(net_param, net_param.asnumpy())
-
- class L1Loss(Loss):
- def __init__(self, reduction="mean", config=True):
- super(L1Loss, self).__init__(reduction)
- self.square = ops.Square()
- self.config = config
-
- def construct(self, base, target):
- x = self.square(base - target)
- return self.get_loss(x)
-
- def get_loss(self, x, weights=1.0):
- print ('The data shape of x is: ', x.shape)
- input_dtype = x.dtype
- x = self.cast(x, ms.common.dtype.float32)
- weights = self.cast(weights, ms.common.dtype.float32)
- x = self.mul(weights, x)
- if self.reduce and self.average:
- x = self.reduce_mean(x, self.get_axis(x))
- if self.reduce and not self.average:
- x = self.reduce_sum(x, self.get_axis(x))
- if self.config:
- x = self.reduce_mean(x, self.get_axis(x))
- weights = self.cast(-1.0, ms.common.dtype.float32)
- x = self.mul(weights, x)
- x = self.cast(x, input_dtype)
- return x
-
- user_loss = L1Loss()
-
- optim = nn.Momentum(net.trainable_params(), learning_rate=0.01, momentum=0.6)
- model = Model(net, user_loss, optim)
-
- epoch = 1
- model.train(epoch, ds_train, callbacks=[LossMonitor(10)], dataset_sink_mode=True)
-
- for net_param in net.trainable_params():
- print(net_param, net_param.asnumpy())
-
- print ('The total time cost is: {}s'.format(time.time() - start_time))
上述代码就是一个简单的案例,这里我们所做的操作,仅仅是把之前均方误差的求和改成了求和之后取负数。还是需要再强调一遍的是,虽然我们定义的函数是非常简单的内容,但是借用这个方法,我们可以更加灵活的去按照自己的设计定义一些定制化的损失函数。上述代码的执行结果如下:
- The data shape of x is:
- (10, 10, 1)
- ...
- The data shape of x is:
- (10, 10, 1)
- epoch: 1 step: 160, loss is -310517200.0
- Parameter (name=fc.weight, shape=(1, 2), dtype=Float32, requires_grad=True) [[-6154.176 667.4569]]
- Parameter (name=fc.bias, shape=(1,), dtype=Float32, requires_grad=True) [-16418.32]
- The total time cost is: 6.681089878082275s
一共打印了160个The data shape of x is...
,这是因为我们在划分输入的数据集的时候,选择了将160个数据划分为每个batch含10个元素的模块,那么一共就有16个batch,又对这16个batch重复10次,那么就是一共有160个batch,计算损失函数时是以batch为单位的,但是如果只是计算求和或者求平均值的话,不管划分多少个batch结果都是一致的。
总结概要
在不同的训练场景中,我们时常需要使用不同的损失函数来衡量一个模型的计算结果的优劣,本文重点介绍了在MindSpore中如何去自定义一个损失函数。基于MindSpore中的Loss类,我们可以通过继承该类后,再重写construct函数和get_loss函数来实现全面自定义的损失函数形式与内容。
版权声明
本文首发链接为:https://www.cnblogs.com/dechinphy/p/msloss.html
作者ID:DechinPhy
更多原著文章请参考:https://www.cnblogs.com/dechinphy/
打赏专用链接:https://www.cnblogs.com/dechinphy/gallery/image/379634.html