决策支持型数据
丰富的技巧和诀窍可以帮助您做出决策。 (An arsenal of tips and tricks to crack decision-making.)
Whether you live on a concrete jungle or not, you are constantly making decisions. In many cases they might be so hardwired that you don’t even think about them. Your brain just push you through it without even reasoning. What we call “experience” can play an important role here: specifically, against an optimal outcome on decision-making; especially when the “experience” does not make room for considering the data.
无论您是否生活在一个具体的丛林中,您都在不断做出决策。 在许多情况下,它们可能是如此僵硬,以至于您甚至都不考虑它们。 您的大脑甚至没有推理就可以推动您通过。 我们所谓的“ 经验 ”可以在这里发挥重要作用:特别是要反对决策的最佳结果; 特别是当“ 体验 ”没有为考虑数据腾出空间时。
The fundamental role of a Data Scientist is to support decision-making based on data. For that to be accomplished a data-driven culture has to be nurtured and that status quo challenged. The barrier to successfully transitioning from a “gut-feeling” type of culture (System 1) to a data-driven type of approach (System 2) is the people.
数据科学家的基本作用是支持基于数据的决策。 为了实现这一目标,必须培育一种数据驱动的文化,并挑战这种现状。 成功地从“ 直觉 ”类型的文化( 系统1 )过渡到以数据驱动的方法( 系统2 )的障碍是人。
Experienced managers and executives tend to believe on their “intuition” to, quite often, make high-stakes decisions. That has to do a lot with many different types of biases that we’ll cover here, but not limited to. But, also with decision making based on emotions, which research has proven to not be recommended.
经验丰富的经理和行政人员倾向于相信自己的“ 直觉 ”,以便经常做出高风险的决策。 这与我们将在此处涵盖但不限于的许多不同类型的偏差有很大关系。 但是,对于基于情绪的决策,事实证明不推荐进行此项研究。
Just to clarify, by executives and managers, we really mean anybody that is in charge of making decisions, regardless of his/her title on the business card.
只是为了澄清,由管理人员和管理人员来形容,实际上是指负责决策的任何人,无论其名片上的头衔如何。
五个最危险的偏见 (The Five Most Dangerous Biases)
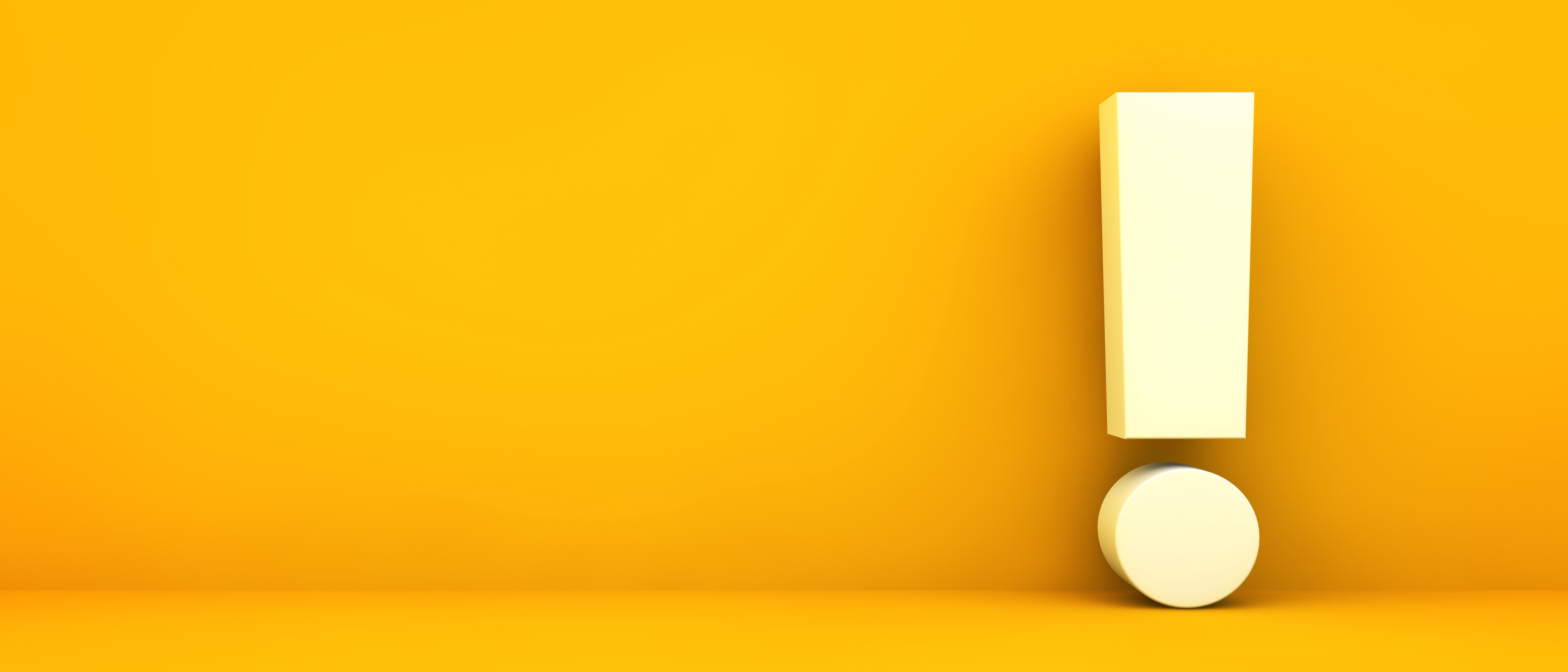
Bias can be defined as, “a particular tendency, trend, inclination, feeling, or opinion, especially one that is preconceived or unreasoned”. Out of the many biases that impact all of us negatively, overconfidence is the worst. Explicitly, when comes down to decision-making processing.
偏差可以定义为“一种特定的趋势,趋势,倾向,感觉或观点,尤其是一种先入为主的或无理的”。 在对我们所有人产生负面影响的许多偏见中, 过度自信是最糟糕的。 明确地讲,归结为决策处理。
“Our gut makes us more vulnerable to cognitive biases such as overconfidence” — Max H. Bazerman
“我们的直觉使我们更容易受到过度自信等认知偏差的影响” — Max H. Bazerman
Decision-makers must acknowledge that they functioning under certain types of biases. Without this realization, it will be extremely difficult to break the wheel of making poor decisions. Managers tend to blame everything else when things go down hill, but take enormous credit when they succeed.
决策者必须承认他们在某些类型的偏见下运作。 没有这种认识,就很难打破糟糕的决定。 当事情跌宕起伏时,管理者往往会责备其他一切,但是当他们成功时,他们会赢得巨大的声誉。
Here I’ll cover a little bit of the main types of biases that can impact decision-making.
在这里,我将介绍一些会影响决策的主要偏见类型。
Overconfidence: Research says that, “overconfidence may be the mother of all decision-making biases” [2]. And that the “excessive faith that you know the truth is one form of overconfidence” [2]. If you are too certain that you are making the best decision, you better be doing that supported by data. Remember, “garbage in, garbage out”. Just because you might be using data, that does not mean you’ll succeed every time.
过度自信:研究表明,“过度自信可能是所有决策偏见的根源” [2]。 而且,“过分相信真相是过度自信的一种形式” [2]。 如果您确定自己做出的决定最好,那么最好在数据支持下进行。 记住, “垃圾进,垃圾出” 。 仅仅因为您可能正在使用数据,并不意味着您每次都会成功。
Confirmation Bias: This is an effort to find a justification to a selective matter. Here we’ll look for anything that can support and confirm our beliefs and values. That is, we are consistently finding excuses to explain our failures without taking responsibilities for them.
确认偏差:这是为选择事项找到理由的努力。 在这里,我们将寻找可以支持和确认我们的信念和价值观的任何事物。 也就是说,我们一直在寻找借口来解释我们的失败而不承担责任。
Unrealistically Positive Views of Self: Typically, this can be seen as a going hand in hand with overconfidence. In this bias, the person has an extremely positive view of him/herself. Specially, people that rely on experience to make decisions, tend to get a very strong positive view of themselves. A pill of humility every morning can be an effective remedy here.
对自我的不切实际的积极看法:通常,这可以被视为与过度自信并驾齐驱。 在这种偏见下,该人对他/她自己有非常积极的看法。 特别是,依靠经验来做决定的人往往会对自己有非常强烈的积极看法。 每天早晨谦卑的药丸可以成为一种有效的治疗方法。
Illusion of Control: Usually, this bias comes attached to high levels of a superstitious mindset. One tend to believe that he/she can control random events by relying on things that they can do. Like, “if I wear a boot, it won’t rain”.
控制幻觉:通常,这种偏见与迷信思维的高水平有关。 人们倾向于认为他/她可以通过依靠随机事件来控制随机事件。 例如,“如果我穿靴子,那不会下雨”。
Self-serving Attributions: I’ve already mentioned that before, but now let’s reframe that again: we tend to take a lot of credit when we succeed, and too little blame when we fail. Generally speaking, an executive will blame a failure to bad lick or other factors beyond the decision maker’s control, but credit their success to their skill or experience.
自我服务的归因:我之前已经提到过,但是现在让我们重新构想一下:成功时,我们往往会获得很多荣誉,而失败时,我们会归咎于太多。 一般而言,高管会责备失败的舔lick行为或决策者无法控制的其他因素,而是将其成功归功于他们的技能或经验。
There are many others types of biases, but I wanted to give you a flavor of what we think to be the main ones when comes to decision-making on a professional setting. In essence, people are inclined to make decisions based on emotions rather than rationally, and this put us straight to a bifurcated roadway.
还有许多其他类型的偏见,但是我想带给您一些我们认为在专业环境中进行决策时最主要的偏见。 从本质上讲,人们倾向于根据情感而不是理性地做出决定,这使我们直接陷入了分歧。
永无止境的战争:系统1和系统2 (The War the Never Ends: System 1 and System 2)
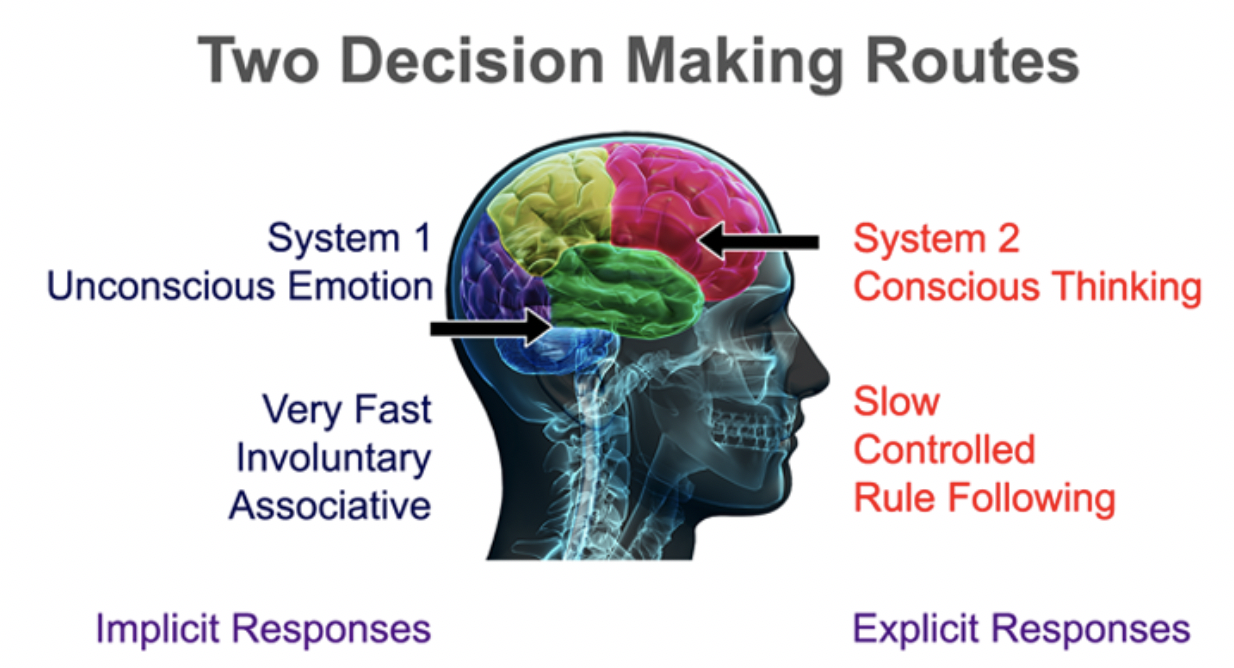
In a nutshell, System 1 relies on emotion, System 2 on rationality. As human beings, we all have both of these systems of thoughts.
简而言之, 系统1依赖于情感, 系统2依赖于理性。 作为人类,我们所有人都有这两种思想体系。
System 1 is based on experience, and requires little cognitive efforts to be utilized. A classic example is the answer to 2 + 2 = ?. You see, you don’t even have to think much in order to find out the right answer. It’s an automatic and very fast processing.
系统1是基于经验的,几乎不需要任何认知努力就可以利用。 一个经典的例子是2 + 2 =?的答案。 您知道,找到正确答案甚至不必花太多时间。 这是一个自动且非常快速的处理。
Likewise, System 2 demands more cognitive effort to be utilized. An example of that is to find the answer to 16 x 23 = ?. For a vast majority of people, finding the correct answer will take more time and more effort. It’s not that automatic anymore.
同样, 系统2需要利用更多的认知努力。 一个例子是找到16 x 23 =?的答案。 对于绝大多数人来说,找到正确的答案将需要更多的时间和精力。 它不再是自动的了。
Why is this relevant for decision-making anyways? It turns out that, when taking important decisions, we want to use System 2, and avoid the pitfalls of System 1. Because, System 1 constantly runs in our brains and never stops — it operates sub-consciously — if System 2 happens to be overloaded — with a lot of many important decisions to make — System 1 will take control and leading you to a decision that, quite often, are not optimal. When this happen we ended up making “good enough” decisions, and increasing the odds of failure.
为什么这与决策相关? 事实证明,在做出重要决策时,我们希望使用系统2,避免使用系统1的陷阱。因为,如果系统2恰好是我们的大脑,那么系统1会不断在我们的大脑中运行,并且不会下意识地停止运行。重载-需要做出许多重要的决定-系统1将控制并引导您做出并非最佳的决定。 当这种情况发生时,我们最终做出“ 足够好 ”的决策,并增加了失败的几率。
规避风险,寻求风险和风险中立 (Risk Averse, Risk Seeking, and Risk Neutral)
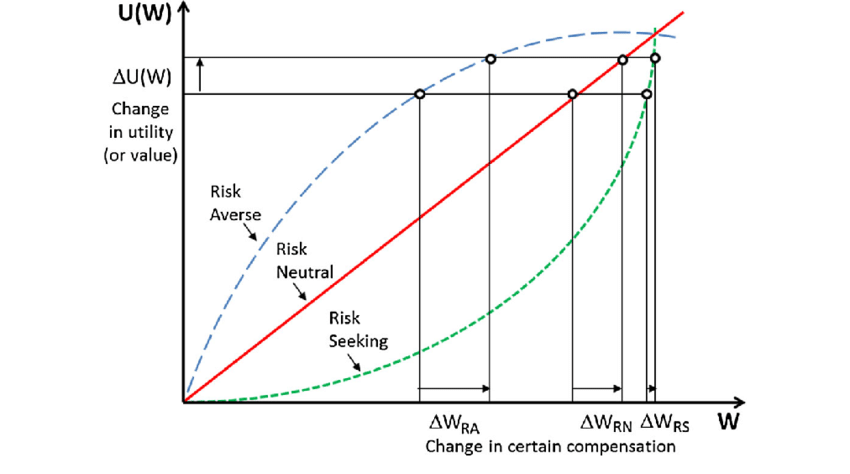
The above chart shows the three different types of approaches when comes down to risk management. This is widely known in Economics and Finance.
上图显示了三种不同类型的风险管理方法。 这在经济学和金融学中是众所周知的。
Well, what this has to do with decision-making? Everything!
那么,这与决策有什么关系? 一切!
People make decisions not only based on monetary value (X axis), but also based on preferences (Y axis), like effort required and/or happiness. This unit of preference is called “utility”, and displays one’s preference for outcomes.
人们不仅基于货币价值(X轴)做出决策,而且还基于偏好 (Y轴)做出决策,例如需要付出的努力和/或幸福。 这个偏好单位称为“ 效用 ”,它显示一个人对结果的偏好。
Utilities are important because they have influence on people’s decisions, and also help us to better understand their attitude towards to risk. In Economics, we see risk as the variance around a mean or expected value. On that regard, we can classify people in these tree categories: Risk Averse, Risk Seeking, and Risk Neutral.
实用程序之所以重要,是因为它们会影响人们的决策,也有助于我们更好地理解他们对风险的态度。 在经济学中,我们将风险视为均值或期望值的方差 。 在这方面,我们可以将人们分类为以下树类:风险规避,寻求风险和风险中立。
Many of us are risk seeking for losses, and risk averse for gains. That is to say, we’ll take a sure thing, but avoid a sure loss. Because losses are more painful than the gains’ pleasure, we want to mitigate them. So, typically, the way executives see “risk” is to be the likelihood and the extent of loss, and not the variance. Hence, they most likely will focus on the downside of risk, and overlook the opportunities. How do you think this can impact decision-making? I’ll leave that to you.
我们中的许多人都在冒险寻求损失,在风险厌恶中寻求收益。 就是说,我们将采取肯定的态度,但要避免必然的损失。 因为损失比获得的乐趣更痛苦,所以我们希望减轻损失。 因此,高管通常认为“风险”的方式是损失的可能性和程度,而不是差异 。 因此,他们极有可能将注意力放在风险的下行方面,而忽视了机会。 您如何看待这会影响决策? 我留给你。
设计更好决策过程的一些工具 (Some Tools to Devise a Better Decision-Making Processing)
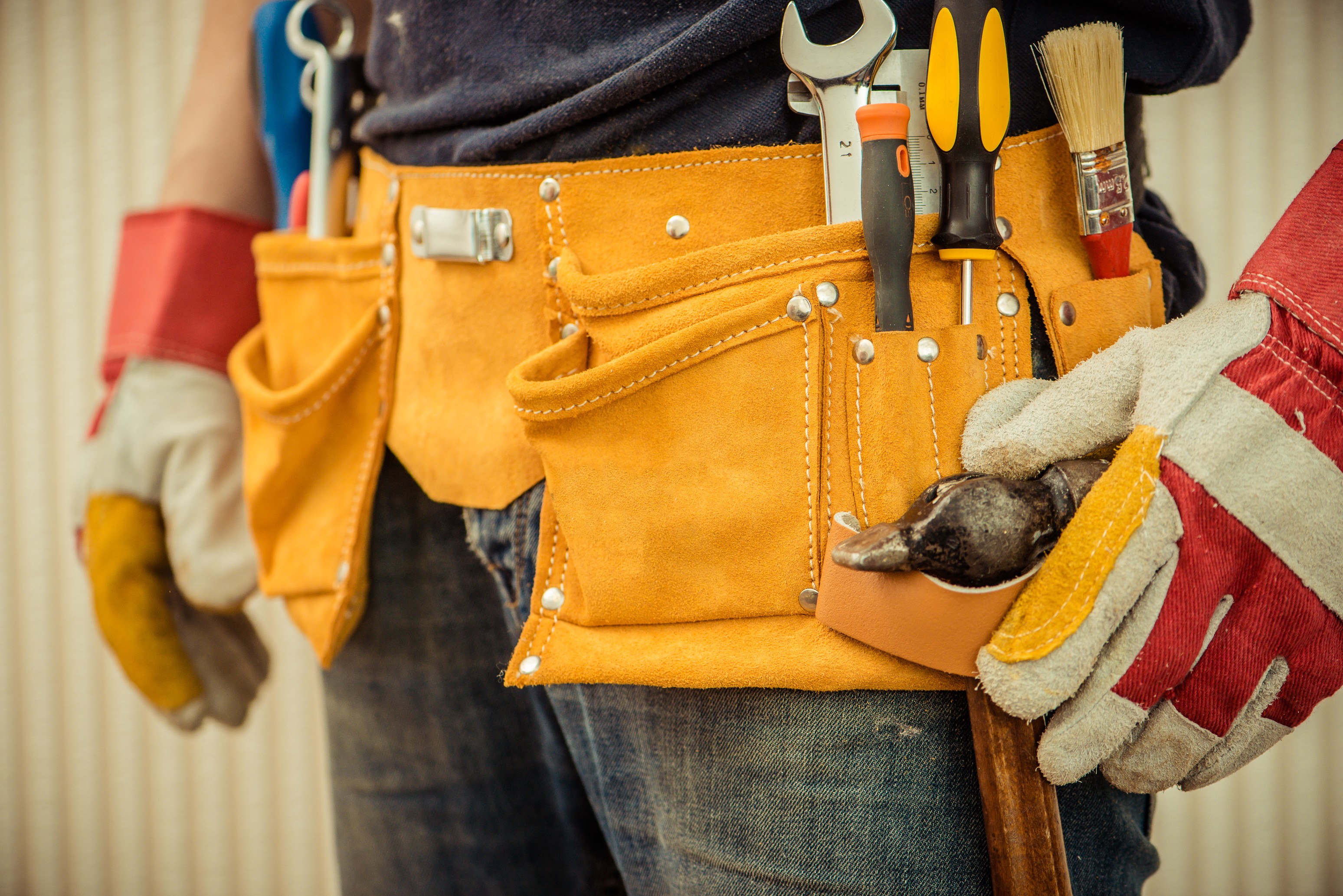
Alright, you gotta have some tools on you belt to help you out, when you are summoned to a meeting you weren’t even aware of.
好了,当您被召集到一次您根本不知道的会议上时,您就可以使用一些工具来帮助您。
Let’s scratch the surface of three basic tools you should know about. Bear in mind that this is not an extensive list.
让我们从头开始,您应该了解三个基本工具。 请记住,这不是详尽的清单。
Decision Table or Decision Matrix
决策表或决策矩阵
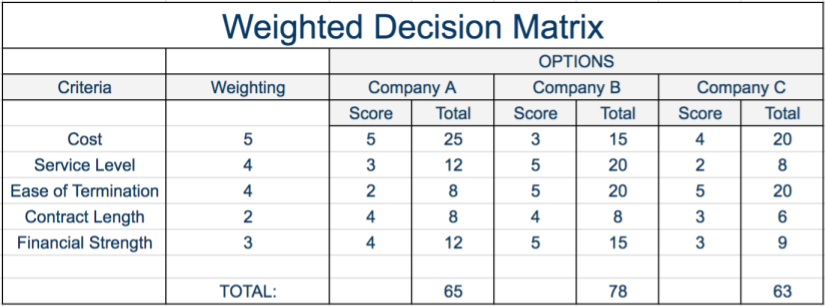
Using a decision table or matrix allows us to come up with a total score that is based on the criteria we’ve selected as most important and the weights we have given to them, according to our preferences (remember the Utility Function?). Although, very basic, the decision table or matrix can be very useful when guiding the decision-maker.
根据我们的偏好 (使用效用函数?),使用决策表或矩阵,我们可以根据我们选择的最重要的标准以及给他们的权重得出总分。 虽然很基本,但是在指导决策者时决策表或矩阵可能非常有用。
2. Decision Trees
2.决策树
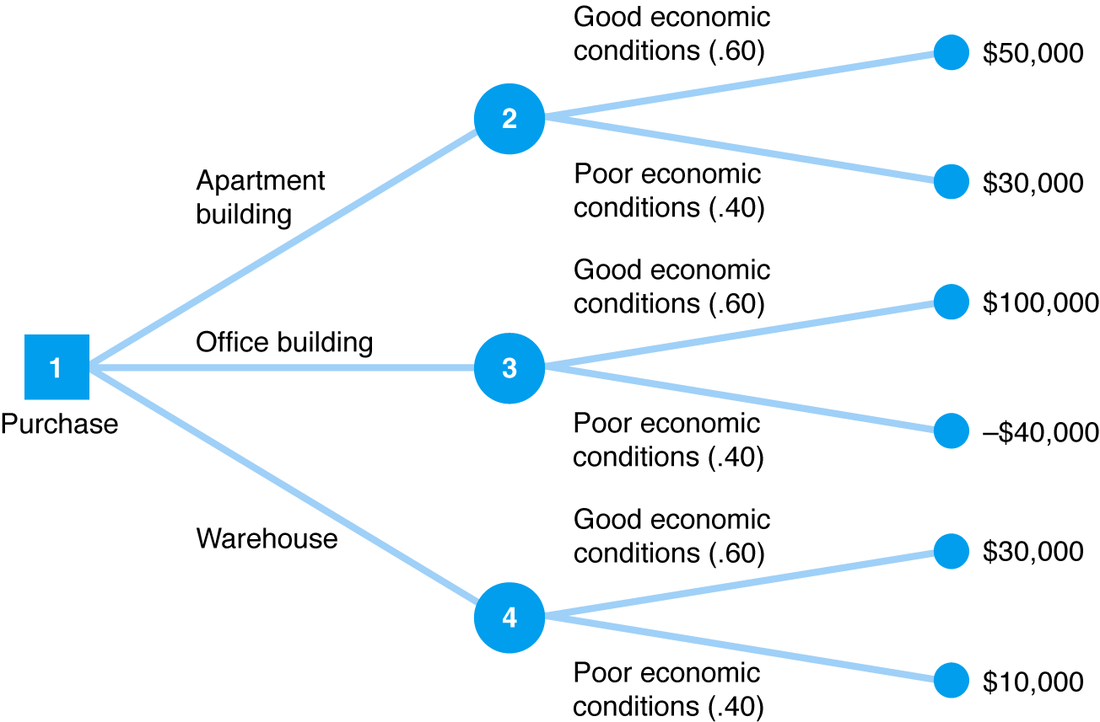
Decision Trees are quite useful to visually the path we are going and the complexity of our decisions as well as the risks and potential outcomes. Machine Learning Engineers and Data Scientist are familiar with decision tree, particularly, because the Decision Trees algorithm can be used in many solutions out there. Check the original article here to learn more.
决策树对于可视化我们要走的道路,决策的复杂性以及风险和潜在结果非常有用。 机器学习工程师和数据科学家特别熟悉决策树,因为决策树算法可用于许多解决方案。 在此处查看原始文章以了解更多信息。
3. Game Theory: Nash Equilibrium
3.博弈论:纳什均衡
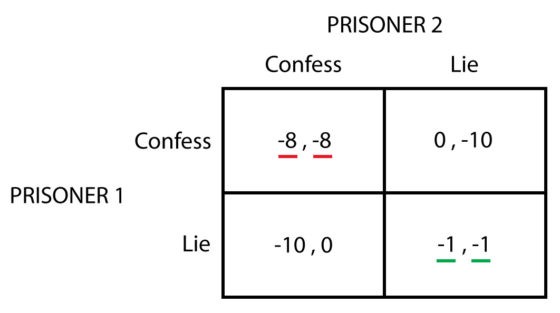
Doing business is to be under the scrutiny of a non-cooperative game. From a business stand point, monopoly would be a dream come true, which means no competition. However, in many industries the competition is fierce. So when companies have to take decisions, the assumption is that they don’t know what their competitors will do. In essence, there is no cooperation when companies are fighting for market share. For that Nash Equilibrium is a great tool. More here.
做生意要受到非合作游戏的审查。 从商业角度来看, 垄断将成为梦想成真,这意味着没有竞争。 但是,在许多行业中竞争是激烈的。 因此,当公司必须做出决定时,假设是他们不知道竞争对手会做什么。 本质上,当公司争夺市场份额时就没有合作。 为此,纳什均衡是一个很好的工具。 这里更多。
数据科学家需要掌握的技能 (Skills a Data Scientist Needs to Master)
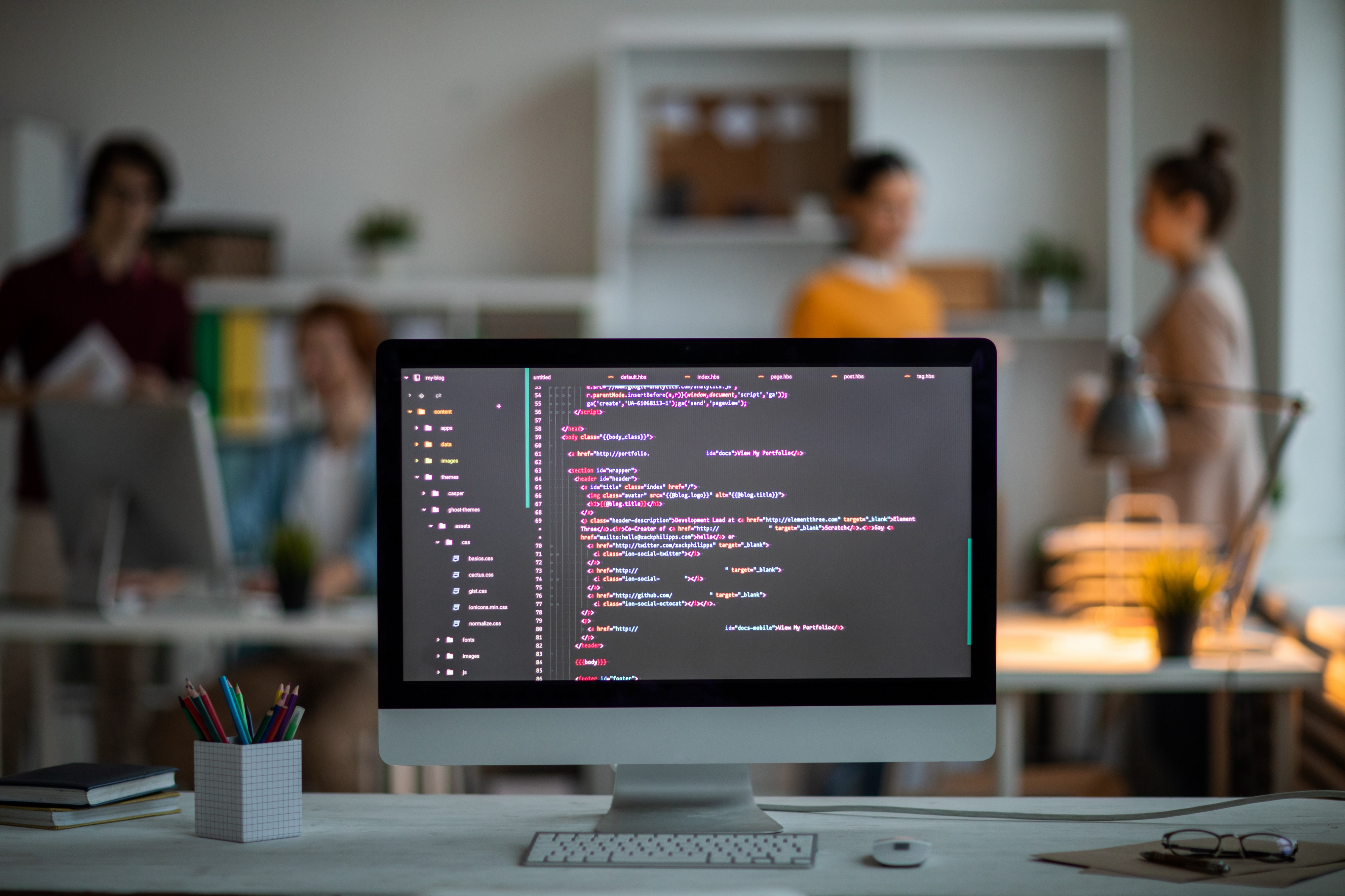
Nope! Your main goal as a DS is not to argue whether or not Python is better or worse than R. This is pointless, and a waste of time.
不! 作为DS的主要目标不是争辩Python是否比R更好或更差。这毫无意义,而且浪费时间。
Rather, your ultimate mission is to support decision-making by fostering a data-driven culture that allows uncertainty to be mitigated, and gut-feeling decision making to become less regurgitated.
相反,您的最终任务是通过培养一种数据驱动的文化来支持决策,这种文化可以减少不确定性,并减少肠胃的决策。
With that in mind, I’ll cover here three soft skills you must work on, if you want to break the wall of resistance when trying to change a culture.
考虑到这一点,如果您想在改变文化时打破阻力,我将在这里介绍您必须研究的三个软技能。
Communication
通讯
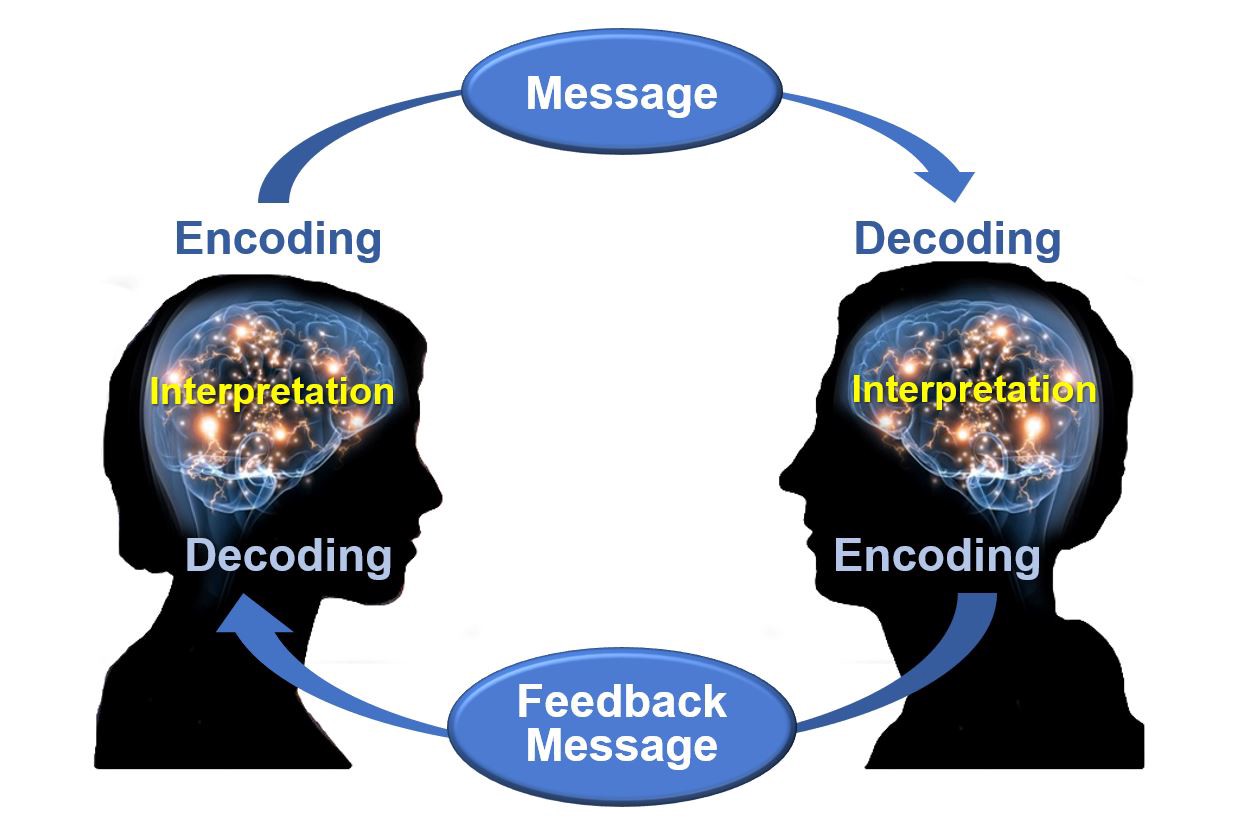
Business people don’t actually care which algorithm you used to come up with your analysis. Stop trying to look smart and to overcomplicate things. Emphasize the solutions you found to their problems, and not the tools you used.
商界人士实际上并不在乎您用来提出分析的算法。 不要试图显得聪明和使事情过于复杂。 强调您找到的解决问题的方法,而不是您使用的工具。
By simplifying things, chances are that you’ll be heard and your message properly decoded. This is important to build trust and rapport because, in general, we like people that look like us.
通过简化事情,很可能会听到您的声音并且正确解码了您的消息。 这对于建立信任和融洽的关系很重要,因为总的来说,我们喜欢长相酷似我们的人。
As data advocates, we want to open the doors for a decision-making process that relies on data. For that, a great communication model is paramount.
作为数据倡导者,我们希望为依赖数据的决策过程打开大门。 为此,出色的沟通模型至关重要。
2. Negotiation Modeling
2.谈判建模
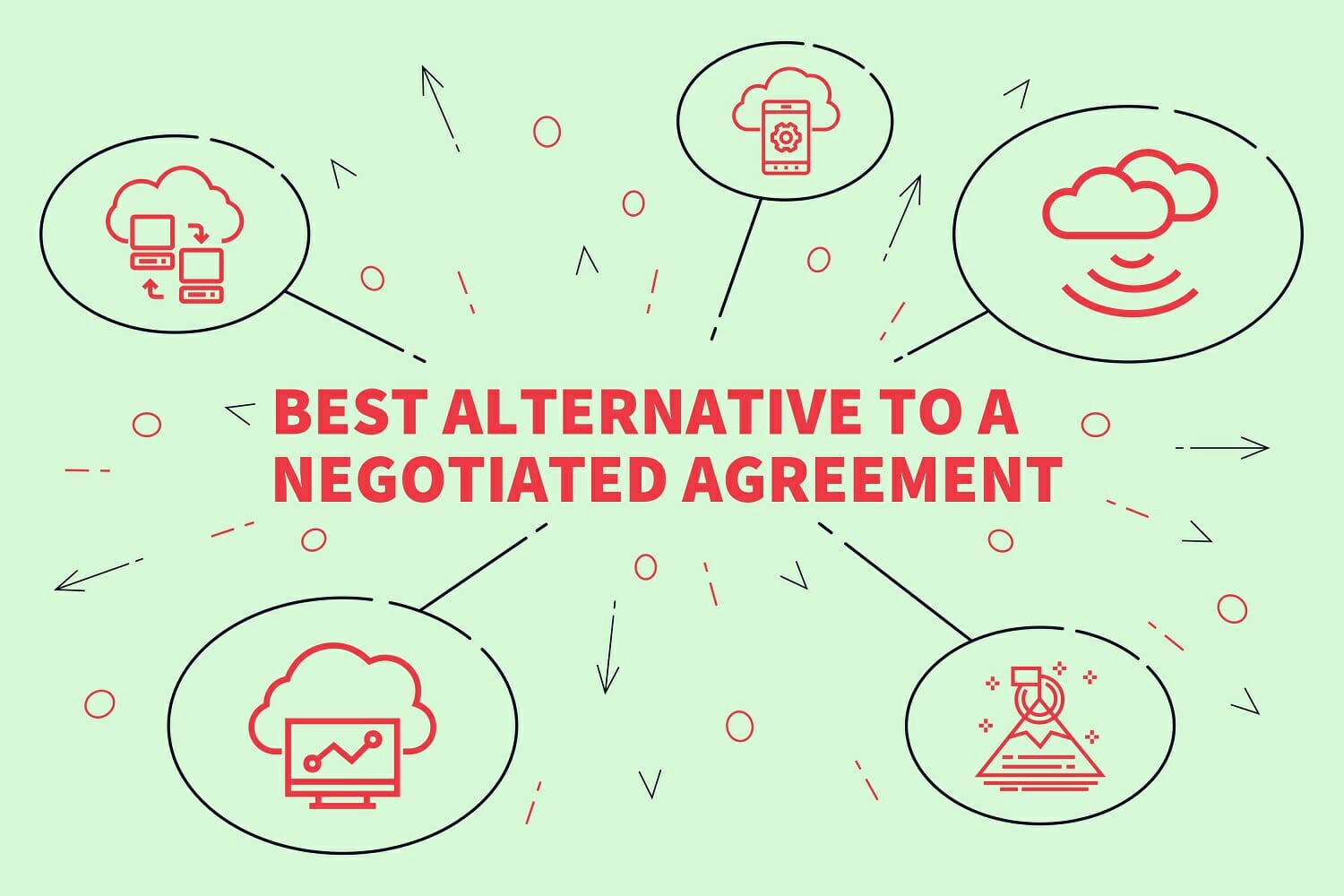
Now, you’ve conveyed your points successfully. But, you find yourself running in circles and trying all you can to implement that, right?! This is where having a knowledge of negotiation can be handy.
现在,您已经成功传达了自己的观点。 但是,您发现自己正在圈子里奔跑,并尽一切可能实现它,对吧? 在这里,可以很容易地掌握谈判知识。
However, don’t put too much effort on winning every battle though. Focus on the war. It’s okay to lose few battles if winning the war is the final goal. If you are just trying to make your point across and prove you’re the smarter person siting on the table, you might fail miserably in the long-run.
但是,尽管赢得每场战斗都不要付出太多努力。 专注于战争。 如果赢得战争是最终目标,则可以输掉几场战斗。 如果您只是想阐明自己的观点,并证明自己是摆在桌子上的聪明人,从长远来看,您可能会惨败。
A great approach is to have a “win-win” attitude. That my friend, can really open the doors. If things got intense, you may want to prepare a good BATNA (Best Alternative To a Negotiated Agreement), which can help you to get what you need without damaging the relationship too much.
一种很好的方法是保持“双赢”的态度。 我的朋友,真的可以打开门。 如果事情变得紧张起来,您可能想准备一个好的BATNA (谈判协议的最佳替代方案),它可以帮助您获得所需的东西而又不会对双方关系造成太大的破坏。
3. Managing Change
3.管理变更
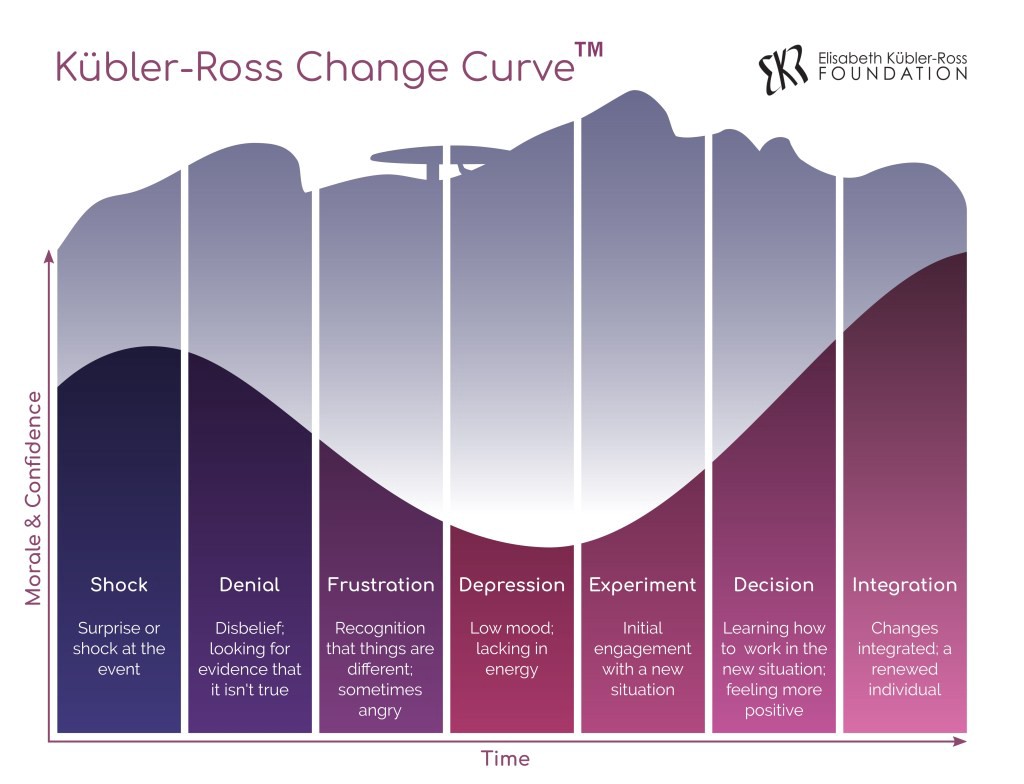
At the end of the day, all boils down to change. And this can be very challenging and it’s more likely to come from a top down decision. Meaning, some higher executive has a data-driven culture and want s to implement that across the firm.
归根结底,一切都归结为变化。 这可能非常具有挑战性,并且更有可能来自自上而下的决策。 这意味着,一些高级管理人员具有数据驱动的文化,并希望在整个公司中实施这种文化。
In general, people tend to be resistant to change. Understanding the steps one goes when dealing with changes is crucial. Remember, creating a data-drive culture is a process and takes time. It’s really a marathon, not a sprint. Be resilient and keep trying to convert and influence others as you navigate towards to a democratization of decision based on data. Persistence is what you’ll need to practice to achieve the integration.
通常,人们倾向于抵制变化。 理解应对变化的第一步至关重要。 记住,创建数据驱动文化是一个过程,需要时间。 这实际上是一场马拉松,而不是短跑。 保持弹性,并在导航到基于数据的决策民主化过程中,不断尝试转变和影响他人。 持久性是实现集成所需的实践。
“Data that is loved tends to survive.” — Kurt Bollacker
“被爱的数据倾向于生存。” — 库尔特·博拉克 ( Kurt Bollacker)
大数据和物联网(IoT) (Big Data and Internet of Things (IoT))
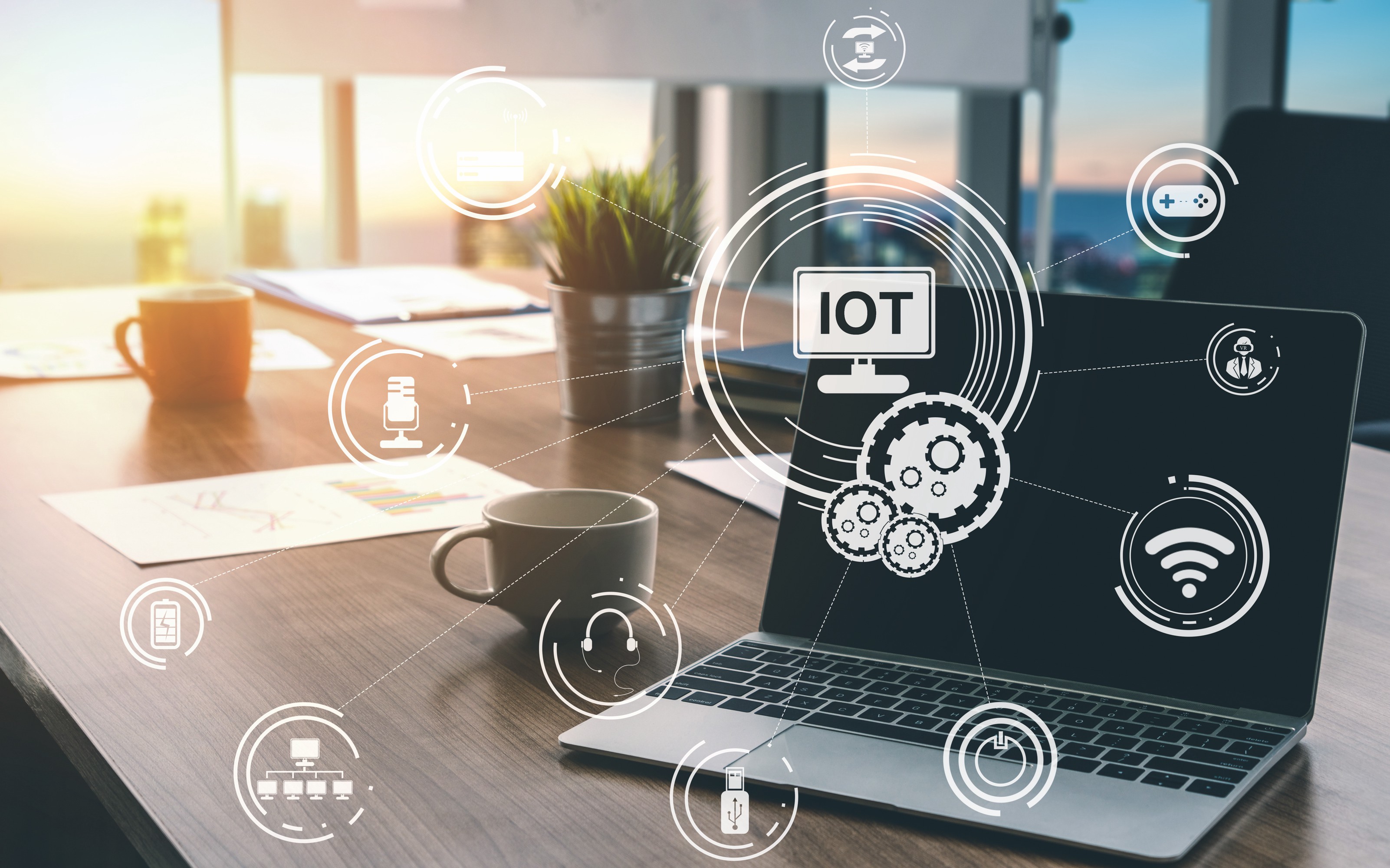
Why decision-makers need Data Science? Simply because, nowadays, the volume and structure of data (Big Data) we have available is immense, and to make sound decisions we need to have data mining capabilities to extract and analyze what matters.
为什么决策者需要数据科学? 很简单,因为,如今,数据量和结构 (大数据),我们有可用的是巨大的,并且使我们需要的数据挖掘能力,提取和分析哪些事项正确的决定。
Think about for a second, today, we have billions of devices connect to the internet 24/7. This generates a lot of data every second. This phenomenon is what we call “Internet of Things” (IoT). Which is directly and positively correlated to the amount to the volume of data we dump in the “big data” world.
想一想,今天,我们有数十亿个设备全天候24/7连接到互联网。 这每秒产生大量数据。 我们称这种现象为“物联网”(IoT)。 这与我们在“大数据”世界中转储的数据量直接相关。
In reality, it’s impossible for a human being successful when analyzing all of that without the machine. The recent advances in hardwire and software has opened windows of opportunities for Data Scientist to thrive and succeed when modeling solutions to companies’ problems.
实际上,在没有机器的情况下分析所有这些信息对于人类来说是不可能的。 硬线和软件方面的最新进展为Data Scientist在为公司问题建模解决方案时蓬勃发展并取得成功提供了机会。
Executives and general leadership cannot close their eyes on what’s happening right now. They must invest on building solid data structures and data warehousing so the analyst can do their job and bring competitive advantage to the table.
高管和一般领导层无法对当前正在发生的事情闭上眼睛。 他们必须投资建立可靠的数据结构和数据仓库,以使分析师能够完成自己的工作并为公司带来竞争优势 。
Making decisions based on when and/or where the wind blows is no longe acceptable.
长期以来,基于风吹的时间和/或位置进行决策是不可接受的。
最后的想法 (Final Thoughts)
All things considered, there are many other topics we didn’t have the chance to cover here. This article aimed to provide a high level of knowledge, and group some resources and information to help you when trying to understand the intricacies of the decision-making process.
考虑到所有因素,还有许多其他主题我们没有机会在这里讨论。 本文旨在提供高水平的知识,并提供一些资源和信息,以帮助您尝试理解决策过程的复杂性。
Technical skills are necessary for the data scientist to perform his/her job, but business knowledge and soft skills are game changing when comes down to nurture changes in the company’s culture towards to a data-driven democratization.
技术技能是数据科学家履行其职责所必需的,但是,当要培养公司文化的变化以实现数据驱动的民主化时,业务知识和软技能正在发生变化。
Here we saw the main biases on the way of decision-making, how System 1 and 2 works, some tools to support decision-making, and some skills a data scientist needs to be successful when fighting status quo.
在这里,我们看到了决策方式的主要偏见,系统1和系统2的工作方式,支持决策的一些工具以及数据科学家在对抗现状时需要成功的一些技能。
I hope this can motivate you to learn more. See you next time.
我希望这可以激励您学习更多。 下次见。
[1] Richard Packard. Why Do I Keep Making Bad Decisions?! BHNW Buddha.
[1]理查德·帕卡德(Richard Packard)。 为什么我会继续做出错误的决定? BHNW佛陀。
[2] Moore, D. A., Tenney, E. R., & Haran, U. (in press). Overprecision in judgment. In G. Wu and G. Keren (Eds.), Handbook of Judgment and Decision Making. New York: Wiley
[2] Moore,DA,Tenney,ER和Haran,U。(印刷中)。 判断中的过分精确。 摘自《判断与决策手册》,G。Wu和G. Keren(编辑)。 纽约:威利
[3] Harris, Christopher & Wu, Chen. (2014). Using tri-reference point theory to evaluate risk attitude and the effects of financial incentives in a gamified crowdsourcing task. Journal of Business Economics. 84. 281–302. 10.1007/s11573–014–0718–4.
[3]哈里斯,克里斯托弗和吴晨。 (2014)。 使用三参考点理论评估游戏化众包任务中的风险态度和财务激励措施。 商业经济学杂志。 84. 281–302。 10.1007 / s11573–014–0718–4。
决策支持型数据