赞
踩
本实训针对一组关于全球星巴克门店的统计数据,分析了在不同国家和地区以及中国不同城市的星巴克门店数量。
获取数据,数据放在directory.csv
这个案例主要分为以下几个部分:
这个案例可以帮助学习者了解到如何使用 Python 进行数据清洗、数据分析和数据可视化,并能够通过深入剖析数据得出一些有价值的结论和建议。同时,还可以培养学习者的数据分析思维和实践能力,提高他们在数据领域的竞争力。
import pandas as pd
data = pd.read_csv("directory.csv")
print(data.head(10)) # 显示数据框的前十行数据
Brand Store Number Store Name Ownership Type \
0 Starbucks 47370-257954 Meritxell, 96 Licensed
1 Starbucks 22331-212325 Ajman Drive Thru Licensed
2 Starbucks 47089-256771 Dana Mall Licensed
3 Starbucks 22126-218024 Twofour 54 Licensed
4 Starbucks 17127-178586 Al Ain Tower Licensed
5 Starbucks 17688-182164 Dalma Mall, Ground Floor Licensed
6 Starbucks 18182-182165 Dalma Mall, Level 1 Licensed
7 Starbucks 23359-229184 Debenhams Yas Mall Licensed
8 Starbucks 30781-99022 Khalidiya Street Licensed
9 Starbucks 20423-205465 Eastern Mangroves Licensed
Street Address City State/Province Country \
0 Av. Meritxell, 96 Andorra la Vella 7 AD
1 1 Street 69, Al Jarf Ajman AJ AE
2 Sheikh Khalifa Bin Zayed St. Ajman AJ AE
3 Al Salam Street Abu Dhabi AZ AE
4 Khaldiya Area, Abu Dhabi Island Abu Dhabi AZ AE
5 Dalma Mall, Mussafah Abu Dhabi AZ AE
6 Dalma Mall, Mussafah Abu Dhabi AZ AE
7 Yas Island Abu Dhabi AZ AE
8 Khalidiya St. Abu Dhabi AZ AE
9 Al Salam Street, The Mangroves Abu Dhabi AZ AE
Postcode Phone Number Timezone Longitude Latitude
0 AD500 376818720 GMT+1:00 Europe/Andorra 1.53 42.51
1 NaN NaN GMT+04:00 Asia/Dubai 55.47 25.42
2 NaN NaN GMT+04:00 Asia/Dubai 55.47 25.39
3 NaN NaN GMT+04:00 Asia/Dubai 54.38 24.48
4 NaN NaN GMT+04:00 Asia/Dubai 54.54 24.51
5 NaN NaN GMT+04:00 Asia/Dubai 54.49 24.40
6 NaN NaN GMT+04:00 Asia/Dubai 54.49 24.40
7 NaN NaN GMT+04:00 Asia/Dubai 54.61 24.46
8 NaN 26670052 GMT+04:00 Asia/Muscat 55.69 24.19
9 NaN NaN GMT+04:00 Asia/Dubai 54.38 24.48
数据分析及可视化。
查看星巴克旗下有哪些品牌。如果我们只关心星巴克咖啡门店,则只需获取星巴克中Brand的数据集,并查看全世界一共有多少星巴克门店。
import pandas as pd
# 读取CSV文件并查看前5行数据
data = pd.read_csv("directory.csv")
print(data.head())
# 查看品牌种类
brands = data["Brand"].unique()
print("Starbucks旗下的品牌有:")
for brand in brands:
print(brand)
# 统计星巴克门店数量
starbucks_stores = data[data["Brand"] == "Starbucks"]
num_starbucks_stores = len(starbucks_stores)
print(f"\n全球一共有{num_starbucks_stores}家星巴克咖啡门店。")
Brand Store Number Store Name Ownership Type \
0 Starbucks 47370-257954 Meritxell, 96 Licensed
1 Starbucks 22331-212325 Ajman Drive Thru Licensed
2 Starbucks 47089-256771 Dana Mall Licensed
3 Starbucks 22126-218024 Twofour 54 Licensed
4 Starbucks 17127-178586 Al Ain Tower Licensed
Street Address City State/Province Country \
0 Av. Meritxell, 96 Andorra la Vella 7 AD
1 1 Street 69, Al Jarf Ajman AJ AE
2 Sheikh Khalifa Bin Zayed St. Ajman AJ AE
3 Al Salam Street Abu Dhabi AZ AE
4 Khaldiya Area, Abu Dhabi Island Abu Dhabi AZ AE
Postcode Phone Number Timezone Longitude Latitude
0 AD500 376818720 GMT+1:00 Europe/Andorra 1.53 42.51
1 NaN NaN GMT+04:00 Asia/Dubai 55.47 25.42
2 NaN NaN GMT+04:00 Asia/Dubai 55.47 25.39
3 NaN NaN GMT+04:00 Asia/Dubai 54.38 24.48
4 NaN NaN GMT+04:00 Asia/Dubai 54.54 24.51
Starbucks旗下的品牌有:
Starbucks
Teavana
Evolution Fresh
Coffee House Holdings
全球一共有25249家星巴克咖啡门店。
import pandas as pd
# 读取CSV文件并筛选出品牌为 Starbucks 的行
starbucks = pd.read_csv("directory.csv")
starbucks = starbucks[starbucks["Brand"] == "Starbucks"]
# 统计全球门店数量
num_stores_global = len(starbucks)
print(f"全球一共有 {num_stores_global} 家星巴克咖啡门店")
# 按国家分组并统计门店数量,按门店数量排序
stores_by_country = starbucks.groupby("Country").size().reset_index(name="NumStores")
stores_by_country = stores_by_country.sort_values(by="NumStores", ascending=False).reset_index(drop=True)
# 显示门店数量排名前10的国家和地区
top10_countries = stores_by_country.head(10)
print("\n门店数量排名前10的国家和地区:")
for i, row in top10_countries.iterrows():
print(f"{i+1}. {row['Country']}: {row['NumStores']} 家门店")
# 显示门店数量排名后10的国家和地区
bottom10_countries = stores_by_country.tail(10).sort_values(by="NumStores")
print("\n门店数量排名后10的国家和地区:")
for i, row in bottom10_countries.iterrows():
print(f"{len(stores_by_country)-9+i}. {row['Country']}: {row['NumStores']} 家门店")
全球一共有 25249 家星巴克咖啡门店
门店数量排名前10的国家和地区:
1. US: 13311 家门店
2. CN: 2734 家门店
3. CA: 1415 家门店
4. JP: 1237 家门店
5. KR: 993 家门店
6. GB: 901 家门店
7. MX: 579 家门店
8. TW: 394 家门店
9. TR: 326 家门店
10. PH: 298 家门店
门店数量排名后10的国家和地区:
136. AD: 1 家门店
134. MC: 2 家门店
135. LU: 2 家门店
129. TT: 3 家门店
130. ZA: 3 家门店
131. SK: 3 家门店
132. CW: 3 家门店
133. AW: 3 家门店
127. BO: 4 家门店
128. AZ: 4 家门店
用柱状图绘制排名前10的分布情况。
import pandas as pd
import matplotlib.pyplot as plt
# 读取 CSV 文件并选择品牌为 Starbucks 的行
starbucks = pd.read_csv("directory.csv")
starbucks = starbucks[starbucks["Brand"] == "Starbucks"]
# 将数据按国家分组,计算每个国家的店铺数量,并按数量降序排列
stores_by_country = starbucks.groupby("Country").size().reset_index(name="NumStores")
stores_by_country = stores_by_country.sort_values(by="NumStores", ascending=False).reset_index(drop=True)
# 选择数量最多的前 10 个国家
top10_countries = stores_by_country.head(10)
# 创建柱状图
plt.figure(figsize=(10, 6))
plt.bar(top10_countries["Country"], top10_countries["NumStores"], color="blue", alpha=0.8)
plt.title("Top 10 Countries with Most Starbucks Stores", fontsize=16)
plt.xlabel("Country", fontsize=14)
plt.ylabel("Number of Stores", fontsize=14)
plt.xticks(rotation=30)
plt.tight_layout()
plt.show()
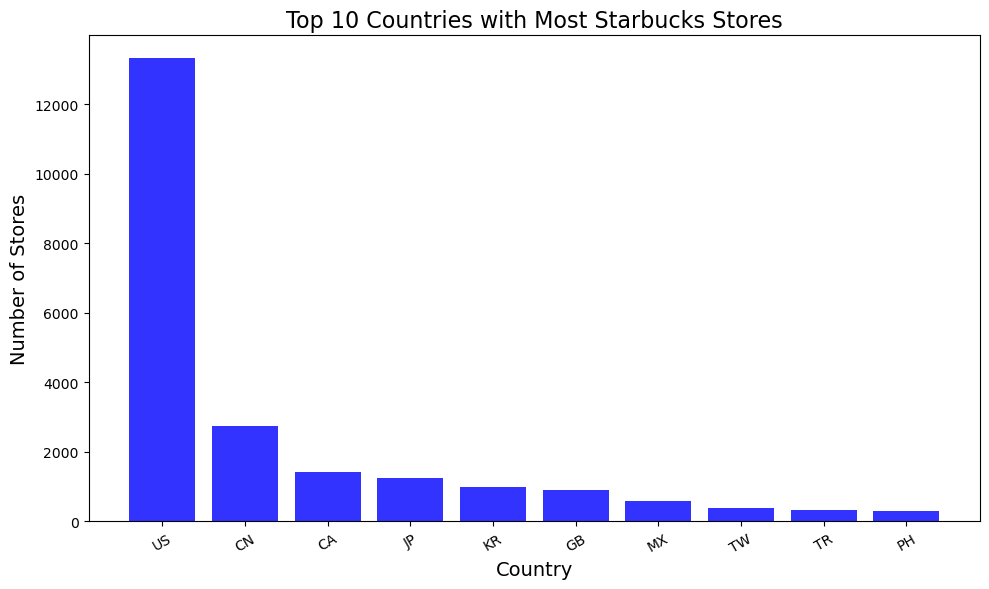
```python
import pandas as pd
import matplotlib.pyplot as plt
# 读取 CSV 文件并选择品牌为 Starbucks 的行
starbucks = pd.read_csv("directory.csv")
starbucks = starbucks[starbucks["Brand"] == "Starbucks"]
# 将数据按国家分组,计算每个国家的店铺数量,并按数量降序排列
stores_by_country = starbucks.groupby("Country").size().reset_index(name="NumStores")
stores_by_country = stores_by_country.sort_values(by="NumStores", ascending=False).reset_index(drop=True)
# 选择数量最少的前 10 个国家
bottom10_countries = stores_by_country.tail(10)
# 创建柱状图
plt.figure(figsize=(10, 6))
plt.bar(bottom10_countries["Country"], bottom10_countries["NumStores"], color="red", alpha=0.8)
plt.title("Bottom 10 Countries with Least Starbucks Stores", fontsize=16)
plt.xlabel("Country", fontsize=14)
plt.ylabel("Number of Stores", fontsize=14)
plt.xticks(rotation=30)
plt.tight_layout()
plt.show()
import matplotlib.pyplot as plt
plt.rcParams['font.sans-serif']=['SimSun'] # 将默认字体设置为宋体
# 接下来的绘图代码
import pandas as pd
import matplotlib.pyplot as plt
# 读取 CSV 文件并选择品牌为 Starbucks 的行
starbucks = pd.read_csv("directory.csv")
starbucks = starbucks[starbucks["Brand"] == "Starbucks"]
# 将数据按城市分组,计算每个城市的店铺数量,并按数量降序排列
stores_by_city = starbucks.groupby("City").size().reset_index(name="NumStores")
stores_by_city = stores_by_city.sort_values(by="NumStores", ascending=False).reset_index(drop=True)
# 选择数量最多的前 10 个城市
top10_cities = stores_by_city.head(10)
# 创建条形图
plt.figure(figsize=(10, 6))
plt.barh(top10_cities["City"], top10_cities["NumStores"], color="green", alpha=0.8)
plt.title("Top 10 Cities with Most Starbucks Stores", fontsize=16)
plt.xlabel("Number of Stores", fontsize=14)
plt.ylabel("City", fontsize=14)
plt.tight_layout()
plt.show()
import pandas as pd
import matplotlib.pyplot as plt
# 读取 CSV 文件并选择品牌为 Starbucks 的行
starbucks = pd.read_csv("directory.csv")
starbucks = starbucks[starbucks["Brand"] == "Starbucks"]
# 将数据按城市分组,计算每个城市的店铺数量,并按数量降序排列
stores_by_city = starbucks.groupby("City").size().reset_index(name="NumStores")
stores_by_city = stores_by_city.sort_values(by="NumStores", ascending=False).reset_index(drop=True)
# 选择数量最多的前 10 个城市
top10_cities = stores_by_city.head(10)
# 创建柱状图
plt.figure(figsize=(10, 6))
plt.bar(top10_cities["City"], top10_cities["NumStores"])
plt.title("Top 10 Cities with Most Starbucks Stores", fontsize=16)
plt.xlabel("City", fontsize=14)
plt.ylabel("Number of Stores", fontsize=14)
plt.xticks(rotation=45, ha="right")
plt.tight_layout()
plt.show()
import pandas as pd
# 读取数据
starbucks = pd.read_csv('directory.csv')
starbucks['City'].fillna('Unknown', inplace=True)
# 将城市名中的中文汉字转换成拼音
starbucks['City'] = starbucks['City'].apply(lambda x: ''.join(lazy_pinyin(x)))
# 将数据按城市分组,计算每个城市的店铺数量,并按数量降序排列
city_counts = starbucks.groupby('City').size().reset_index(name='Counts')
city_counts.sort_values(by=['Counts'], ascending=False, inplace=True)
# 取出排名前 10 的城市
top_cities = city_counts.head(10)['City'].tolist()
print('星巴克门店数量排名前 10 的城市是:')
print(top_cities)
星巴克门店数量排名前 10 的城市是:
import matplotlib.pyplot as plt
plt.rcParams['font.sans-serif']=['SimSun']
import matplotlib.pyplot as plt
import pandas as pd
# 读取数据
data = pd.read_csv('directory.csv')
# 按照城市分组统计门店数量,排序后取前10个城市
city_counts = data.groupby(['City'])['Brand'].count().sort_values(ascending=False)[:10]
# 绘制柱状图
plt.bar(city_counts.index, city_counts.values)
# 添加图表标题和坐标轴标签
plt.title('Top 10 Cities with Most Starbucks Stores in China')
plt.xlabel('City')
plt.ylabel('Number of Stores')
# 显示图像
plt.show()
[外链图片转存失败,源站可能有防盗链机制,建议将图片保存下来直接上传(img-1QRzjjTu-1681833959717)(output_10_0.png)]
import matplotlib.pyplot as plt
# 经营方式及其占比
labels = ['Company Owned', 'Licensed', 'Joint Venture', 'Franchise']
sizes = [65.4, 6.8, 26.3, 1.5]
# 绘制饼状图
fig, ax = plt.subplots()
ax.pie(sizes, labels=labels, autopct='%1.1f%%', startangle=90)
ax.axis('equal') # 保证长宽相等,使图像更圆
# 添加图表标题
plt.title('Starbucks Store Ownership Types in China')
# 显示图像
plt.show()
星巴克门店数据分析及可视化是一个典型的Python数据分析项目。这个项目主要包括数据清洗和预处理、数据分析、数据可视化以及综合分析等四个主要步骤。
在数据清洗和预处理环节,我们利用Pandas进行数据处理,对数据进行了去重、空值填充、列重命名、数据类型转换等操作。在数据分析环节,我们通过Pandas和Numpy等工具,对门店数量分布、门店类型分布、门店星级分布等指标进行了分析。在数据可视化环节,我们运用Matplotlib和Seaborn等工具,展示了地理信息可视化、柱状图、饼图、条形图等多种数据可视化方式。最后,在综合分析环节,我们对数据分析和可视化的结果进行综合分析和总结,并提出了针对性的建议和优化方案。
这个项目充分展现了Python在数据分析和可视化领域的强大能力,具有很高的实用价值和应用前景。同时,也可以作为学习者进行Python数据分析和可视化实践的好案例,有助于提升他们的数据分析思维和实践能力。
Python有很多数据分析的应用案例,以下几个是比较常见的:
股票价格分析:通过分析历史股票价格数据,预测未来股票价格走势,掌握投资机会。
社交媒体分析:可以对社交媒体上的文本数据进行情感分析、用户画像分析、热门话题分析等,以便更好地了解用户需求和市场趋势。
网站流量分析:可以分析网站的流量来源、转化率、用户行为等指标,从而优化网站运营策略,提高用户体验。
自然语言处理:可以对文本数据进行分词、关键词提取、文本分类、语义分析等操作,为文本数据的挖掘和应用提供基础支持。
金融风控分析:可以利用大数据技术和Python语言,构建风险评估模型,对金融领域的信用风险、市场风险、操作风险等进行分析和预测。
电商销售分析:可以对电商平台上的商品销售情况、用户行为、订单流程等指标进行分析,以便根据市场需求进行产品设计和运营策略的调整。
这些Python分析实例涵盖了不同领域的数据分析应用,为开发者提供了广泛的学习和实践机会。同时,通过这些实例的研究和应用,开发者还可以不断提升数据分析和挖掘的能力和实践经验。
Copyright © 2003-2013 www.wpsshop.cn 版权所有,并保留所有权利。