赞
踩
先自我介绍一下,小编浙江大学毕业,去过华为、字节跳动等大厂,目前阿里P7
深知大多数程序员,想要提升技能,往往是自己摸索成长,但自己不成体系的自学效果低效又漫长,而且极易碰到天花板技术停滞不前!
因此收集整理了一份《2024年最新网络安全全套学习资料》,初衷也很简单,就是希望能够帮助到想自学提升又不知道该从何学起的朋友。
既有适合小白学习的零基础资料,也有适合3年以上经验的小伙伴深入学习提升的进阶课程,涵盖了95%以上网络安全知识点,真正体系化!
由于文件比较多,这里只是将部分目录截图出来,全套包含大厂面经、学习笔记、源码讲义、实战项目、大纲路线、讲解视频,并且后续会持续更新
如果你需要这些资料,可以添加V获取:vip204888 (备注网络安全)
self.label_1.setText(_translate("MainWindow", "识别结果:"))
self.label_2.setText(_translate("MainWindow", "车牌区域:"))
self.label_3.setText(_translate("MainWindow", ""))
self.pushButton.setText(_translate("MainWindow", "打开文件"))
self.pushButton_2.setText(_translate("MainWindow", "导出数据"))
self.label_4.setText(_translate("MainWindow", "事件:"))
self.scrollAreaWidgetContents_1.show()
UI实现效果如下: 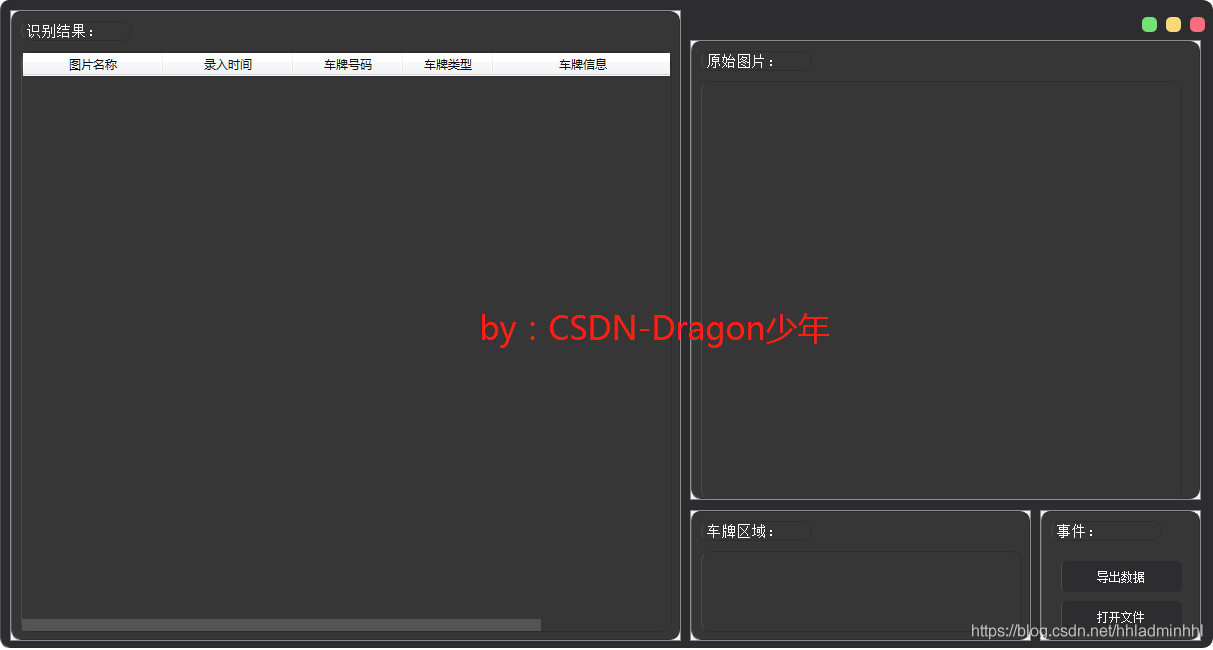 ##### 2. 车牌识别 接下来我们需要实现两个核心功能,包括获取**车牌ROI区域**和**车牌自动识别**功能。 **车牌ROI区域提取:** 根据读取的车辆图片,预处理进行车牌ROI区域提取,主要通过Opencv的图像处理相关知识点来完成。主要包括对图像去噪、二值化、边缘轮廓提取、矩形区域矫正、蓝绿黄车牌颜色定位识别。核心代码如下:
def pretreatment(self, car_pic):
if type(car_pic) == type(“”):
img = self.__imreadex(car_pic)
else:
img = car_pic
pic_hight, pic_width = img.shape[:2]
if pic_width > self.MAX_WIDTH: resize_rate = self.MAX_WIDTH / pic_width img = cv2.resize(img, (self.MAX_WIDTH, int(pic_hight \* resize_rate)), interpolation=cv2.INTER_AREA) # 图片分辨率调整 blur = self.cfg["blur"] # 高斯去噪 if blur > 0: img = cv2.GaussianBlur(img, (blur, blur), 0) oldimg = img img = cv2.cvtColor(img, cv2.COLOR_BGR2GRAY) kernel = np.ones((20, 20), np.uint8) img_opening = cv2.morphologyEx(img, cv2.MORPH_OPEN, kernel) # 开运算 img_opening = cv2.addWeighted(img, 1, img_opening, -1, 0); # 与上一次开运算结果融合 # cv2.imshow('img\_opening', img\_opening) # 找到图像边缘 ret, img_thresh = cv2.threshold(img_opening, 0, 255, cv2.THRESH_BINARY + cv2.THRESH_OTSU) # 二值化 img_edge = cv2.Canny(img_thresh, 100, 200) # cv2.imshow('img\_edge', img\_edge) # 使用开运算和闭运算让图像边缘成为一个整体 kernel = np.ones((self.cfg["morphologyr"], self.cfg["morphologyc"]), np.uint8) img_edge1 = cv2.morphologyEx(img_edge, cv2.MORPH_CLOSE, kernel) # 闭运算 img_edge2 = cv2.morphologyEx(img_edge1, cv2.MORPH_OPEN, kernel) # 开运算 # cv2.imshow('img\_edge2', img\_edge2) # cv2.imwrite('./edge2.png', img\_edge2) # 查找图像边缘整体形成的矩形区域,可能有很多,车牌就在其中一个矩形区域中 image, contours, hierarchy = cv2.findContours(img_edge2, cv2.RETR_TREE, cv2.CHAIN_APPROX_SIMPLE) contours = [cnt for cnt in contours if cv2.contourArea(cnt) > self.Min_Area] # 逐个排除不是车牌的矩形区域 car_contours = [] for cnt in contours: # 框选 生成最小外接矩形 返回值(中心(x,y), (宽,高), 旋转角度) rect = cv2.minAreaRect(cnt) # print('宽高:',rect[1]) area_width, area_height = rect[1] # 选择宽大于高的区域 if area_width < area_height: area_width, area_height = area_height, area_width wh_ratio = area_width / area_height # print('宽高比:',wh\_ratio) # 要求矩形区域长宽比在2到5.5之间,2到5.5是车牌的长宽比,其余的矩形排除 if wh_ratio > 2 and wh_ratio < 5.5: car_contours.append(rect) box = cv2.boxPoints(rect) box = np.int0(box) # 矩形区域可能是倾斜的矩形,需要矫正,以便使用颜色定位 card_imgs = [] for rect in car_contours: if rect[2] > -1 and rect[2] < 1: # 创造角度,使得左、高、右、低拿到正确的值 angle = 1 else: angle = rect[2] rect = (rect[0], (rect[1][0] + 5, rect[1][1] + 5), angle) # 扩大范围,避免车牌边缘被排除 box = cv2.boxPoints(rect) heigth_point = right_point = [0, 0] left_point = low_point = [pic_width, pic_hight] for point in box: if left_point[0] > point[0]: left_point = point if low_point[1] > point[1]: low_point = point if heigth_point[1] < point[1]: heigth_point = point if right_point[0] < point[0]: right_point = point if left_point[1] <= right_point[1]: # 正角度 new_right_point = [right_point[0], heigth_point[1]] pts2 = np.float32([left_point, heigth_point, new_right_point]) # 字符只是高度需要改变 pts1 = np.float32([left_point, heigth_point, right_point]) M = cv2.getAffineTransform(pts1, pts2) dst = cv2.warpAffine(oldimg, M, (pic_width, pic_hight)) self.__point_limit(new_right_point) self.__point_limit(heigth_point) self.__point_limit(left_point) card_img = dst[int(left_point[1]):int(heigth_point[1]), int(left_point[0]):int(new_right_point[0])] card_imgs.append(card_img) elif left_point[1] > right_point[1]: # 负角度 new_left_point = [left_point[0], heigth_point[1]] pts2 = np.float32([new_left_point, heigth_point, right_point]) # 字符只是高度需要改变 pts1 = np.float32([left_point, heigth_point, right_point]) M = cv2.getAffineTransform(pts1, pts2) dst = cv2.warpAffine(oldimg, M, (pic_width, pic_hight)) self.__point_limit(right_point) self.__point_limit(heigth_point) self.__point_limit(new_left_point) card_img = dst[int(right_point[1]):int(heigth_point[1]), int(new_left_point[0]):int(right_point[0])] card_imgs.append(card_img) #使用颜色定位,排除不是车牌的矩形,目前只识别蓝、绿、黄车牌 colors = [] for card_index, card_img in enumerate(card_imgs): green = yellow = blue = black = white = 0 try: # 有转换失败的可能,原因来自于上面矫正矩形出错 card_img_hsv = cv2.cvtColor(card_img, cv2.COLOR_BGR2HSV) except: print('BGR转HSV失败') card_imgs = colors = None return card_imgs, colors if card_img_hsv is None: continue row_num, col_num = card_img_hsv.shape[:2] card_img_count = row_num \* col_num # 确定车牌颜色 for i in range(row_num): for j in range(col_num): H = card_img_hsv.item(i, j, 0) S = card_img_hsv.item(i, j, 1) V = card_img_hsv.item(i, j, 2) if 11 < H <= 34 and S > 34: # 图片分辨率调整 yellow += 1 elif 35 < H <= 99 and S > 34: # 图片分辨率调整 green += 1 elif 99 < H <= 124 and S > 34: # 图片分辨率调整 blue += 1 if 0 < H < 180 and 0 < S < 255 and 0 < V < 46: black += 1 elif 0 < H < 180 and 0 < S < 43 and 221 < V < 225: white += 1 color = "no" # print('黄:{:<6}绿:{:<6}蓝:{:<6}'.format(yellow,green,blue)) limit1 = limit2 = 0 if yellow \* 2 >= card_img_count: color = "yellow" limit1 = 11 limit2 = 34 # 有的图片有色偏偏绿 elif green \* 2 >= card_img_count: color = "green" limit1 = 35 limit2 = 99 elif blue \* 2 >= card_img_count: color = "blue" limit1 = 100 limit2 = 124 # 有的图片有色偏偏紫 elif black + white >= card_img_count \* 0.7: color = "bw" # print(color) colors.append(color) # print(blue, green, yellow, black, white, card\_img\_count) if limit1 == 0: continue # 根据车牌颜色再定位,缩小边缘非车牌边界 xl, xr, yh, yl = self.accurate_place(card_img_hsv, limit1, limit2, color) if yl == yh and xl == xr: continue need_accurate = False if yl >= yh: yl = 0 yh = row_num need_accurate = True if xl >= xr: xl = 0 xr = col_num need_accurate = True card_imgs[card_index] = card_img[yl:yh, xl:xr] \ if color != "green" or yl < (yh - yl) // 4 else card_img[yl - (yh - yl) // 4:yh, xl:xr] if need_accurate: # 可能x或y方向未缩小,需要再试一次 card_img = card_imgs[card_index] card_img_hsv = cv2.cvtColor(card_img, cv2.COLOR_BGR2HSV) xl, xr, yh, yl = self.accurate_place(card_img_hsv, limit1, limit2, color) if yl == yh and xl == xr: continue if yl >= yh: yl = 0 yh = row_num if xl >= xr: xl = 0 xr = col_num card_imgs[card_index] = card_img[yl:yh, xl:xr] \ if color != "green" or yl < (yh - yl) // 4 else card_img[yl - (yh - yl) // 4:yh, xl:xr] # cv2.imshow("result", card\_imgs[0]) # cv2.imwrite('1.jpg', card\_imgs[0]) # print('颜色识别结果:' + colors[0]) return card_imgs, colors
至此我们就可以输出车牌ROI区域和车牌颜色了,效果如下: 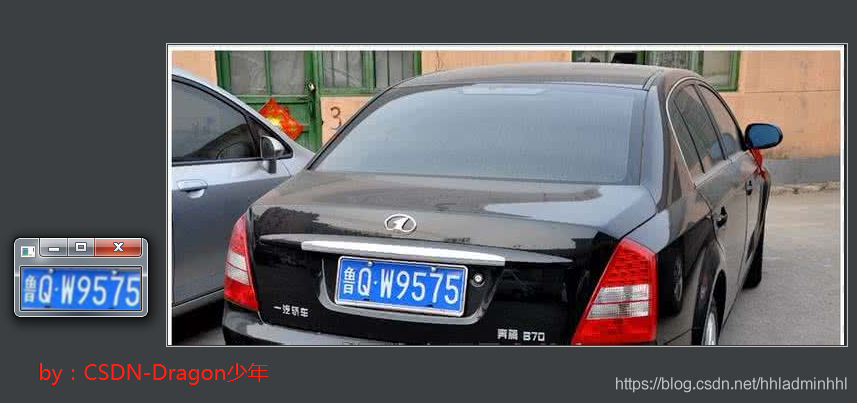 **车牌自动识别:** 车牌识别博主自己写了一个基于Opencv和SVM的识别系统,由于代码篇幅较长,本篇不进行展示(**感兴趣的可以私信博主获取源码**)。本篇介绍调用百度AI提供的车牌识别接口 – [百度AI开放平台链接]( ),识别效果也非常不错。 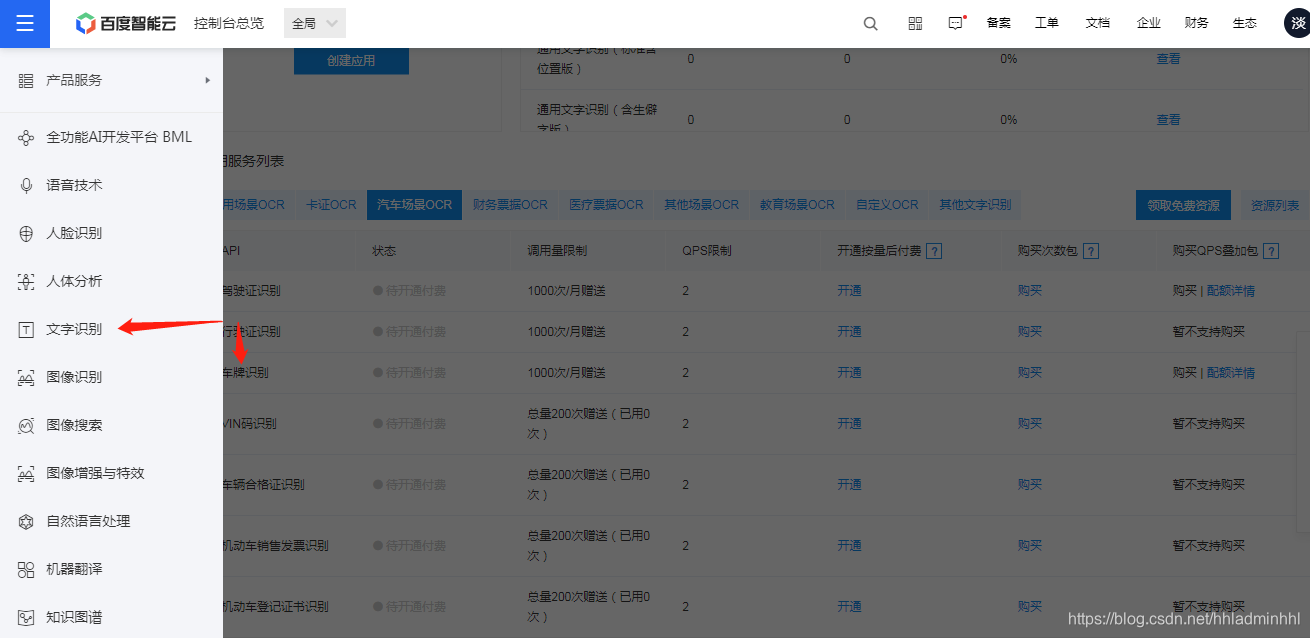 这里面我们可以创建一个车牌识别的应用,其中的**API Key及Secret Key**后面我们调用车牌识别检测接口时会用到。  我们可以看到官方提供的帮助文档,介绍了如何**调用请求URL数据格式,向API服务地址使用POST发送请求**,必须在URL中带上参数**access\_token**,可通过后台的API Key和Secret Key生成。这里面的API Key和Secret Key就是我们上面提到的。 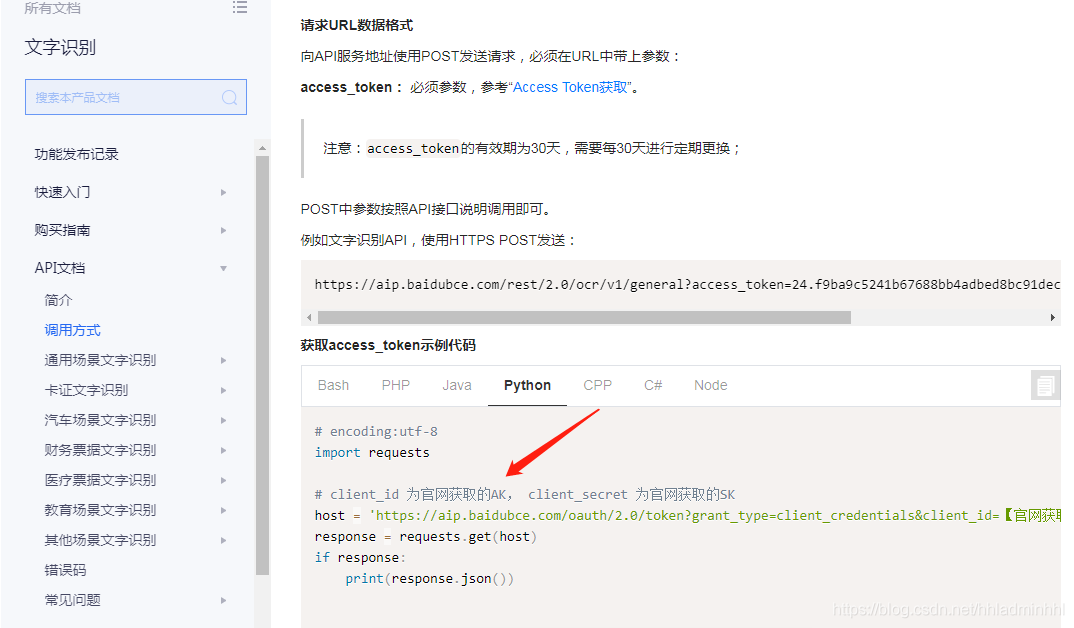 接下来我们看看调用车牌识别接口代码示例。 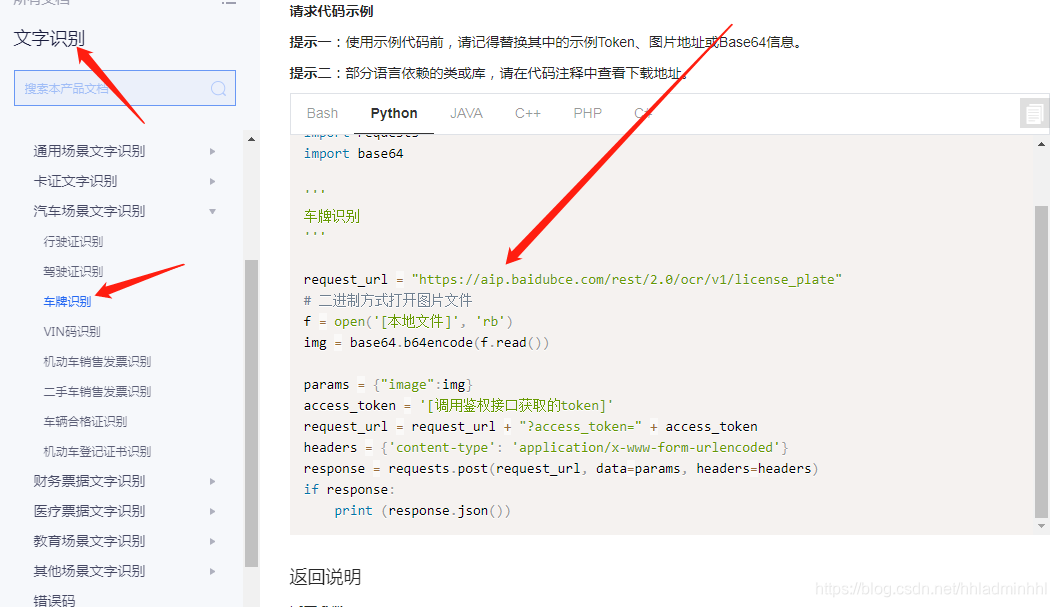 那我们如何获取识别的车牌号码呢?API文档可以看到里面有个**words\_result字典** ,其中的**color代表车牌颜色** ,**number代表车牌号码** 。这样我就可以知道识别的车牌颜色和车牌号了。 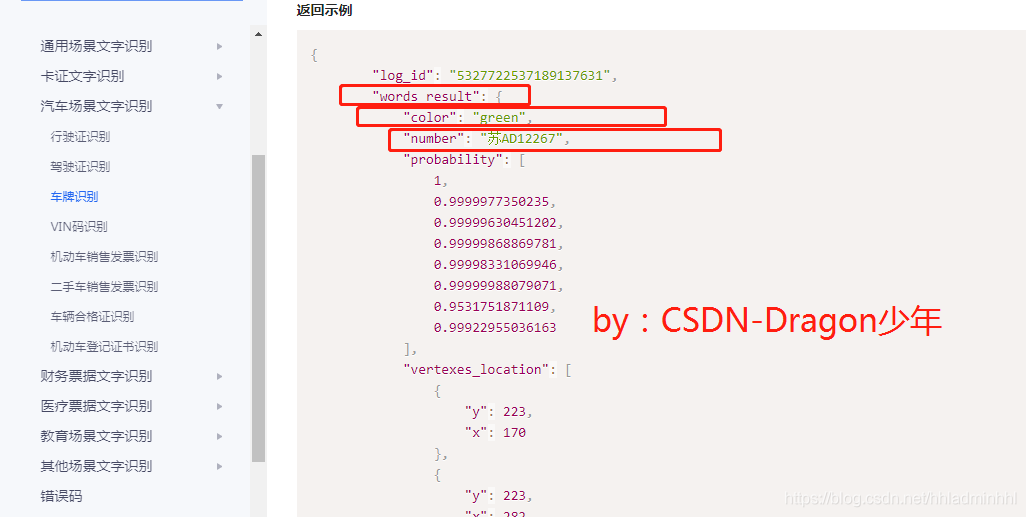 车牌识别的接口调用流程基本已经清楚了,下面就可以进行代码实现了。
def get_token(self):
host = ‘https://aip.baidubce.com/oauth/2.0/token?grant_type=client_credentials&client_id=’ + self.client_id + ‘&client_secret=’ + self.client_secret
response = requests.get(host)
if response:
token_info = response.json()
token_key = token_info[‘access_token’]
return token_key
def get_license_plate(self, car_pic):
result = {}
card_imgs, colors = self.pretreatment(car_pic)
request_url = “https://aip.baidubce.com/rest/2.0/ocr/v1/license_plate”
# 二进制方式打开图片文件
f = open(car_pic, ‘rb’)
img = base64.b64encode(f.read())
params = {“image”: img}
access_token = self.get_token()
request_url = request_url + “?access_token=” + access_token
headers = {‘content-type’: ‘application/x-www-form-urlencoded’}
response = requests.post(request_url, data=params, headers=headers)
if response:
print(response.json())
license_result = response.json()[‘words_result’][‘number’]
card_color = response.json()[‘words_result’][‘color’]
if license_result != []:
result[‘InputTime’] = time.strftime(“%Y-%m-%d %H:%M:%S”)
result[‘Type’] = self.cardtype[card_color]
result[‘Picture’] = card_imgs[0]
result[‘Number’] = ‘’.join(license_result[:2]) + ‘·’ + ‘’.join(license_result[2:])
try:
result[‘From’] = ‘’.join(self.Prefecture[license_result[0]][license_result[1]])
except:
result[‘From’] = ‘未知’
return result
else:
return None
这样我们就可以拿到车牌颜色和车牌号码了,效果如下:
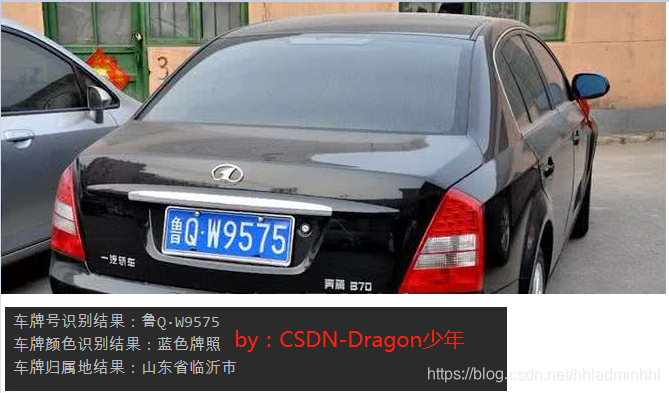
##### 3. 车牌信息显示存储
###### 3.1 车牌信息显示:
def __show(self, result, FileName):
# 显示表格
self.RowLength = self.RowLength + 1
if self.RowLength > 18:
self.tableWidget.setColumnWidth(5, 157)
self.tableWidget.setRowCount(self.RowLength)
self.tableWidget.setItem(self.RowLength - 1, 0, QTableWidgetItem(FileName))
self.tableWidget.setItem(self.RowLength - 1, 1, QTableWidgetItem(result[‘InputTime’]))
self.tableWidget.setItem(self.RowLength - 1, 2, QTableWidgetItem(result[‘Number’]))
self.tableWidget.setItem(self.RowLength - 1, 3, QTableWidgetItem(result[‘Type’]))
if result[‘Type’] == ‘蓝色牌照’:
self.tableWidget.item(self.RowLength - 1, 3).setBackground(QBrush(QColor(3, 128, 255)))
elif result[‘Type’] == ‘绿色牌照’:
self.tableWidget.item(self.RowLength - 1, 3).setBackground(QBrush(QColor(98, 198, 148)))
elif result[‘Type’] == ‘黄色牌照’:
self.tableWidget.item(self.RowLength - 1, 3).setBackground(QBrush(QColor(242, 202, 9)))
self.tableWidget.setItem(self.RowLength - 1, 4, QTableWidgetItem(result[‘From’]))
self.tableWidget.item(self.RowLength - 1, 4).setBackground(QBrush(QColor(255, 255, 255)))
# 显示识别到的车牌位置
size = (int(self.label_3.width()), int(self.label_3.height()))
shrink = cv2.resize(result[‘Picture’], size, interpolation=cv2.INTER_AREA)
shrink = cv2.cvtColor(shrink, cv2.COLOR_BGR2RGB)
self.QtImg = QtGui.QImage(shrink[:], shrink.shape[1], shrink.shape[0], shrink.shape[1] * 3,
QtGui.QImage.Format_RGB888)
self.label_3.setPixmap(QtGui.QPixmap.fromImage(self.QtImg))
效果如下:
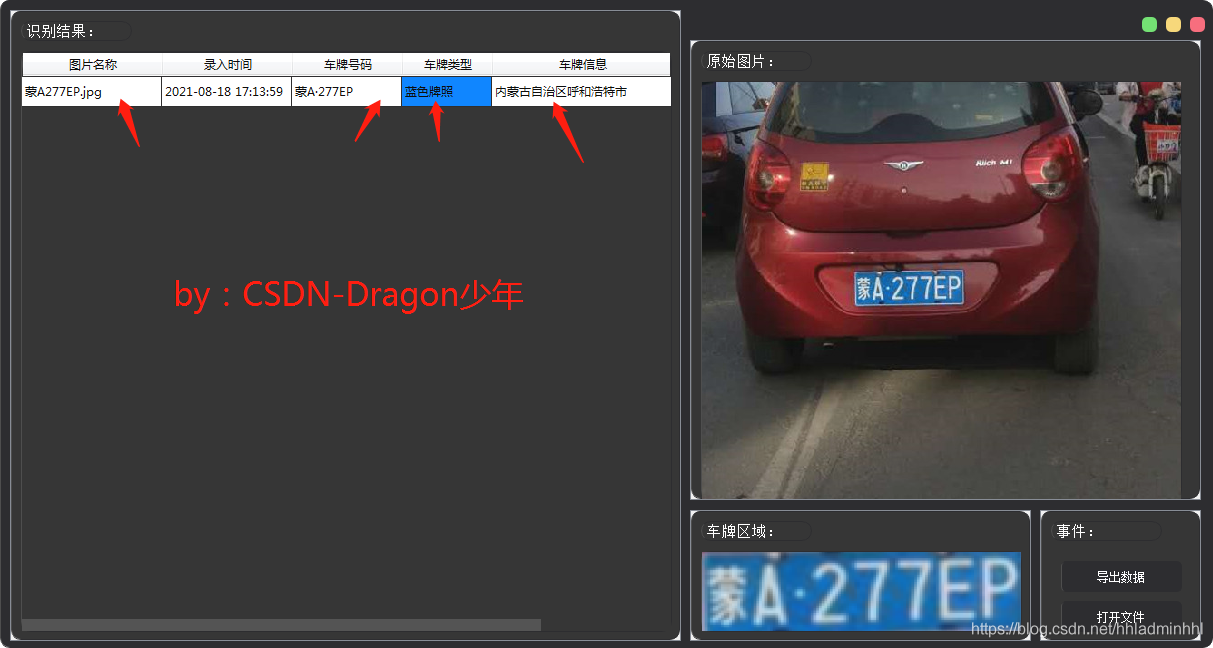
###### 3.2 信息导出存储:
def __writexls(self, DATA, path):
wb = xlwt.Workbook();
ws = wb.add_sheet(‘Data’);
# DATA.insert(0, [‘文件名称’,‘录入时间’, ‘车牌号码’, ‘车牌类型’, ‘车牌信息’])
for i, Data in enumerate(DATA):
for j, data in enumerate(Data):
ws.write(i, j, data)
wb.save(path)
QMessageBox.information(None, “成功”, “数据已保存!”, QMessageBox.Yes)
def __writecsv(self, DATA, path):
f = open(path, ‘w’)
# DATA.insert(0, [‘文件名称’,‘录入时间’, ‘车牌号码’, ‘车牌类型’, ‘车牌信息’])
for data in DATA:
f.write((‘,’).join(data) + ‘\n’)
f.close()
QMessageBox.information(None, “成功”, “数据已保存!”, QMessageBox.Yes)
def __writeFiles(self):
path, filetype = QFileDialog.getSaveFileName(None, “另存为”, self.ProjectPath,
“Excel 工作簿(*.xls);;CSV (逗号分隔)(*.csv)”)
if path == “”: # 未选择
return
if filetype == ‘Excel 工作簿(*.xls)’:
self.__writexls(self.Data, path)
elif filetype == ‘CSV (逗号分隔)(*.csv)’:
self.__writecsv(self.Data, path)
## 写在最后 **在结束之际,我想重申的是,学习并非如攀登险峻高峰,而是如滴水穿石般的持久累积。尤其当我们步入工作岗位之后,持之以恒的学习变得愈发不易,如同在茫茫大海中独自划舟,稍有松懈便可能被巨浪吞噬。然而,对于我们程序员而言,学习是生存之本,是我们在激烈市场竞争中立于不败之地的关键。一旦停止学习,我们便如同逆水行舟,不进则退,终将被时代的洪流所淘汰。因此,不断汲取新知识,不仅是对自己的提升,更是对自己的一份珍贵投资。让我们不断磨砺自己,与时代共同进步,书写属于我们的辉煌篇章。** 需要完整版PDF学习资源私我 **网上学习资料一大堆,但如果学到的知识不成体系,遇到问题时只是浅尝辄止,不再深入研究,那么很难做到真正的技术提升。** **需要这份系统化的资料的朋友,可以添加V获取:vip204888 (备注网络安全)** 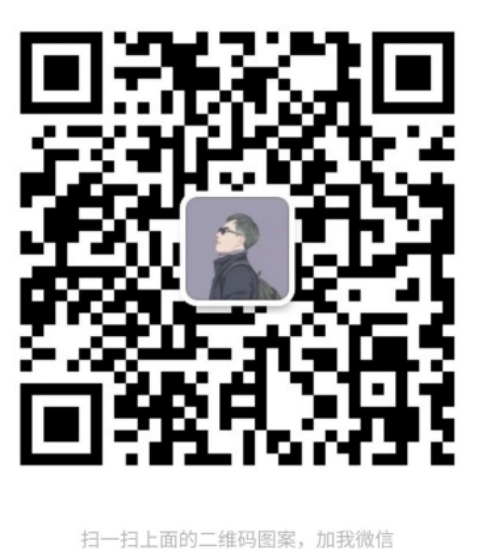 **一个人可以走的很快,但一群人才能走的更远!不论你是正从事IT行业的老鸟或是对IT行业感兴趣的新人,都欢迎加入我们的的圈子(技术交流、学习资源、职场吐槽、大厂内推、面试辅导),让我们一起学习成长!** 激烈市场竞争中立于不败之地的关键。一旦停止学习,我们便如同逆水行舟,不进则退,终将被时代的洪流所淘汰。因此,不断汲取新知识,不仅是对自己的提升,更是对自己的一份珍贵投资。让我们不断磨砺自己,与时代共同进步,书写属于我们的辉煌篇章。** 需要完整版PDF学习资源私我 **网上学习资料一大堆,但如果学到的知识不成体系,遇到问题时只是浅尝辄止,不再深入研究,那么很难做到真正的技术提升。** **需要这份系统化的资料的朋友,可以添加V获取:vip204888 (备注网络安全)** [外链图片转存中...(img-LIMProPB-1713478285994)] **一个人可以走的很快,但一群人才能走的更远!不论你是正从事IT行业的老鸟或是对IT行业感兴趣的新人,都欢迎加入我们的的圈子(技术交流、学习资源、职场吐槽、大厂内推、面试辅导),让我们一起学习成长!**
Copyright © 2003-2013 www.wpsshop.cn 版权所有,并保留所有权利。