熵权法
熵值法的主要目的是对指标体系进行赋权
熵越大说明系统越混乱,携带的信息越少,权重越小;熵越小说明系统越有序,携带的信息越多,权重越大。
熵值法是一种客观赋权方法,,借鉴了信息熵思想,它通过计算指标的信息熵,根据指标的相对变化程度对系统整体的影响来决定指标的权重,即根据各个指标标志值的差异程度来进行赋权,从而得出各个指标相应的权重,相对变化程度大的指标具有较大的权重。
收集与整理
假设有m个待评价样本,n项评价指标,形成原始指标数据矩阵:
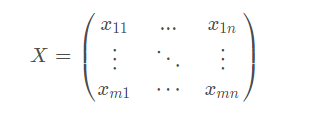
其中Xij表示第 i 个样本第 j 项评价指标的数值。
对于某项指标Xj,样本的离散程度越大,则该指标在综合评价中所起的作用就越大。如果该指标的标志值全部相等,则表示该指标在综合评价中不起作用。
数据标准化处理
为消除因量纲不同对评价结果的影响,需要对各指标进行标准化处理。
若所用指标的值越大越好(正向指标:)
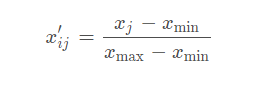
若所用指标的值越小越好(负向指标:)
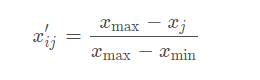
其中xj为第j项指标值, xmax为第j项指标的最大值, xmin为第j项指标的最小值。或者:
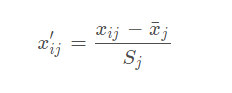
权重计算
计算第 j 个指标中,第 i 个样本标志值的权重:
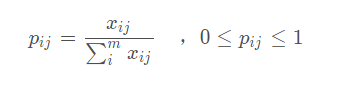
因此,可以建立数据的比重矩阵
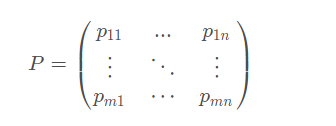
计算第j个指标的熵值
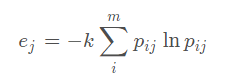
其中,常数
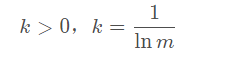
保证0<=ej<=1,即最大为1
所以,第j个指标的熵值为
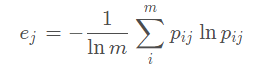
定义第j个指标的差异程度
熵值法根据各个指标标志值的差异程度来进行赋权,从而得出各个指标相应的权重
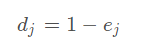
定义权重
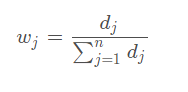
综合评价
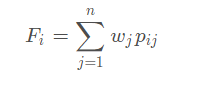
原理讲解引自:https://blog.csdn.net/qq_42374697/article/details/105901229
题目
评价下表中20条河流的水质情况。(熵权法和优劣解距离法对比)
注:含氧量越高越好;PH值越接近7越好;细菌总数越少越好;植物性营养物量介于10‐20之间最佳,超过20或低于10均不好。
河流 | 含氧量(ppm) | PH值 | 细菌总数(个/mL) | 植物性营养物量(ppm) |
A | 4.69 | 6.59 | 51 | 11.94 |
B | 2.03 | 7.86 | 19 | 6.46 |
C | 9.11 | 6.31 | 46 | 8.91 |
D | 8.61 | 7.05 | 46 | 26.43 |
E | 7.13 | 6.5 | 50 | 23.57 |
F | 2.39 | 6.77 | 38 | 24.62 |
G | 7.69 | 6.79 | 38 | 6.01 |
H | 9.3 | 6.81 | 27 | 31.57 |
I | 5.45 | 7.62 | 5 | 18.46 |
J | 6.19 | 7.27 | 17 | 7.51 |
K | 7.93 | 7.53 | 9 | 6.52 |
L | 4.4 | 7.28 | 17 | 25.3 |
M | 7.46 | 8.24 | 23 | 14.42 |
N | 2.01 | 5.55 | 47 | 26.31 |
O | 2.04 | 6.4 | 23 | 17.91 |
P | 7.73 | 6.14 | 52 | 15.72 |
Q | 6.35 | 7.58 | 25 | 29.46 |
R | 8.29 | 8.41 | 39 | 12.02 |
S | 3.54 | 7.27 | 54 | 3.16 |
T | 7.44 | 6.26 | 8 | 28.41 |
熵权法
.mat数据:在MATLAB里面随便创建一个变量,将表格中的数据粘贴进变量中,再另存为.mat数据就行。
main.m
- %% 数据读取
- clear,clc
- load rivers_data.mat
- %% 正向化处理
- [n,m] = size(datas_matrix);
- % 正向化处理的数据所在列
- Pos = [2,3,4];
- % 指标类型:1:极小型,2:中间型,3:区间型
- ch = [2,1,3];
- % 循环处理每一列
- for i = 1 : size(Pos,2)
- datas_matrix(:,Pos(i)) = Forward_processing(datas_matrix(:,Pos(i)),ch(i),Pos(i));
- end
-
- %% 矩阵标准化
- datas_S_matrix = datas_matrix ./ repmat(sum(datas_matrix.*datas_matrix) .^ 0.5, n, 1);
-
- %%
- model = ["A","B","C","D","E","F","G","H","I","J","K","L","M","N","O","P","Q","R","S","T"];
- %% 熵权法
- p = datas_S_matrix./sum(datas_S_matrix);
- k = 1/log(n);
- r = zeros(n,m);
- for i = 1:n
- for j = 1:m
- if p(i,j) == 0
- r(i,j) = 0;
- else
- r(i,j) = log(p(i,j));
- end
- end
- end
- e = -k*sum(p.*r,1);
- d = ones(1,m)-e;
- weight = d./sum(d);
- score = sum(weight.*datas_S_matrix,2);
- results1 = 0 + (100-0)/(max(score)-min(score)).*(score - min(score));
- [sorted_score,index] = sort(results1 ,'descend');
- rivers1 = [];
- for i = 1:n
- rivers1 = [rivers1;model(index(i))];
- end
- s = [rivers1,sorted_score];
-
-
- %% 绘图
- bar(sorted_score);
- title('熵权法')
- set(gca,'XTick',1:20)
- set(gca, 'xticklabel',{rivers1{1:20}});
-
- %% 保存到文件
- xlswrite('output.xls',s,'Sheet1');
Forward_processing.m
- function [posit_x] = Forward_processing(x,type,~)
- if type == 1 %极小型
- %正向化
- posit_x = max(x) - x;
- elseif type == 2 %中间型
- best = 7;
- M = max(abs(x-best));
- posit_x = 1 - abs(x-best) / M;
- elseif type == 3 %区间型
- a = 10;
- b = 20;
- r_x = size(x,1);
- M = max([a-min(x),max(x)-b]);
- posit_x = zeros(r_x,1);
- for i = 1: r_x
- if x(i) < a
- posit_x(i) = 1-(a-x(i))/M;
- elseif x(i) > b
- posit_x(i) = 1-(x(i)-b)/M;
- else
- posit_x(i) = 1;
- end
- end
- end
- end