赞
踩
文章仅用于记录自己的学习,如有侵权立删
https://github.com/jcjohnson/pytorch-examples pytorch的一些小项目
https://pytorch.org/tutorials/ pytorch官方教程
Fastai
Michael Nielsen的《Neural Network and Deep Learning》(《神经网络与深度学习》) 这个是有关数学原理的一些推导,只是学习pytorch的话可以不用
英文版在线:http://neuralnetworksanddeeplearning.com/about.html
中文版:
Stephen Boyd和Lieven Vandenberghe编写的《Convex Optimaization(凸优化)》
3Blue1Brown 视频讲解有关数学等知识 https://space.bilibili.com/88461692
以下是pytorch官方tutorials的一些学习
https://pytorch.org/tutorials/ 原文见 下面是自己的一些翻译和学习
Authors: Suraj Subramanian, Seth Juarez, Cassie Breviu, Dmitry Soshnikov, Ari Bornstein
大多数机器学习工作流都涉及处理数据、创建模型、优化模型参数和保存经过训练的模型。接下来我们跟着教程学习一下pytorch,随后在minst数据集上进行实验
有两种学习方式,一种是在云上的notebook运行样例代码
Each section has a “Run in Microsoft Learn” link at the top 点击就可以
另一种就是在本地部署环境
PyTorch 有两种处理数据的语句
torch.utils.data.DataLoader
和 torch.utils.data.Dataset
.
Dataset
存储样本及其相应的标签
DataLoader
将dataset封装为一个iterable(迭代器接口)
import torch
from torch import nn
from torch.utils.data import DataLoader
from torchvision import datasets
from torchvision.transforms import ToTensor, Lambda, Compose
import matplotlib.pyplot as plt
PyTorch提供特定于领域的库,如TorchText、TorchVision和TorchAudio,所有这些库都包含数据集。在本教程中,我们将使用TorchVision数据集。
torchvision.datasets
module 包含很多数据集比如coco,cifra
每个TorchVision数据集包含两个参数:transform和target_transform,分别用于修改样本和标签。
例子
# Download training data from open datasets.
training_data = datasets.FashionMNIST(
root="data",
train=True,
download=True,
transform=ToTensor(),
)
# Download test data from open datasets.
test_data = datasets.FashionMNIST(
root="data",
train=False,
download=True,
transform=ToTensor(),
)
我们将数据集作为参数传递给DataLoader。这在我们的数据集上包装了一个iterable,并支持自动批处理、采样、混排和多进程数据加载。在这里,我们定义了64个批量大小,即dataloader iterable中的每个元素将返回一批64个特性和标签。
batch_size = 64
# Create data loaders.
train_dataloader = DataLoader(training_data, batch_size=batch_size)
test_dataloader = DataLoader(test_data, batch_size=batch_size)
for X, y in test_dataloader:
print("Shape of X [N, C, H, W]: ", X.shape)
print("Shape of y: ", y.shape, y.dtype)
break
注:从上面我们可以学习到pytorch读取数据集的逻辑,同时可以理解了pytorch处理数据集的逻辑(即变为一个iterable)下面考虑如何创建模型
为了在PyTorch中定义神经网络,我们创建了一个从nn.Module继承的类。我们在_init__函数中定义网络的层,并在forward函数中指定数据将如何通过网络。为了加速神经网络中的操作,我们将其移动到GPU(如果可用)。
# Get cpu or gpu device for training. device = "cuda" if torch.cuda.is_available() else "cpu" print(f"Using {device} device") # Define model class NeuralNetwork(nn.Module): def __init__(self): super(NeuralNetwork, self).__init__() self.flatten = nn.Flatten() self.linear_relu_stack = nn.Sequential( nn.Linear(28*28, 512), nn.ReLU(), nn.Linear(512, 512), nn.ReLU(), nn.Linear(512, 10) ) def forward(self, x): x = self.flatten(x) logits = self.linear_relu_stack(x) return logits model = NeuralNetwork().to(device) print(model)
关于这个网络结构的解读放到后面
网络结构确定以后我们需要考虑如何训练得到我们需要的网络参数,为此我们需要定义 loss function 和 optimizer
loss_fn = nn.CrossEntropyLoss()
optimizer = torch.optim.SGD(model.parameters(), lr=1e-3)
第一句话 交叉熵损失
第二句话SGD优化器
在单个训练循环中,模型对训练数据集进行预测(分批输入),并反向传播预测误差以调整模型参数。
def train(dataloader, model, loss_fn, optimizer): size = len(dataloader.dataset) model.train() for batch, (X, y) in enumerate(dataloader): X, y = X.to(device), y.to(device) # Compute prediction error pred = model(X)# 输出为预测的输出 loss = loss_fn(pred, y)# 计算loss # Backpropagation 反向传播算法 optimizer.zero_grad()# 梯度置零 loss.backward() optimizer.step() if batch % 100 == 0: loss, current = loss.item(), batch * len(X) print(f"loss: {loss:>7f} [{current:>5d}/{size:>5d}]")
这个仔细看一下具体代码,配合前面的modelue看,结合写的注释后面再具体讲解
def test(dataloader, model, loss_fn):
size = len(dataloader.dataset)
num_batches = len(dataloader)
model.eval()
test_loss, correct = 0, 0
with torch.no_grad():
for X, y in dataloader:
X, y = X.to(device), y.to(device)
pred = model(X)
test_loss += loss_fn(pred, y).item()
correct += (pred.argmax(1) == y).type(torch.float).sum().item()
test_loss /= num_batches
correct /= size
print(f"Test Error: \n Accuracy: {(100*correct):>0.1f}%, Avg loss: {test_loss:>8f} \n")
训练过程在多次迭代(epoch)中进行。 在每个epoch,模型学习参数以做出更好的预测。 我们在每个epoch打印模型的准确性和损失; 我们希望看到准确率随着每个 epoch 的增加而减少。
epochs = 5
for t in range(epochs):
print(f"Epoch {t+1}\n-------------------------------")
train(train_dataloader, model, loss_fn, optimizer)
test(test_dataloader, model, loss_fn)
print("Done!")
torch.save(model.state_dict(), "model.pth")
print("Saved PyTorch Model State to model.pth")
保存模型的操作
model = NeuralNetwork()
model.load_state_dict(torch.load("model.pth"))
读取模型的操作,第一章到此就结束了 下一章介绍tensors
这一章主要介绍tensor的概念
官方解释 Tensors are a specialized data structure that are very similar to arrays and matrices.
张量是一种特殊的数据结构,与数组和矩阵非常相似。 可以理解为pytorch中存储结构,我们使用张量来编码模型的输入和输出,以及模型的参数。
张量类似于 NumPy 的 ndarray,不同之处在于张量可以在 GPU 或其他硬件加速器上运行。张量和 NumPy 数组通常可以共享相同的底层内存,从而无需复制数据
import torch
import numpy as np
Initializing a Tensor初始化tensor/创建一个tensor
从data(矩阵形式)导入tensor
data = [[1, 2],[3, 4]]
x_data = torch.tensor(data)
从numpy数组导入tensor
np_array = np.array(data)
x_np = torch.from_numpy(np_array)
从别的tensor导入(除非明确覆盖,否则新张量保留参数张量的属性(形状、数据类型))
x_ones = torch.ones_like(x_data)
# retains the properties of x_data保留 x_data 的数据类型
print(f"Ones Tensor: \n {x_ones} \n")
x_rand = torch.rand_like(x_data, dtype=torch.float)
# overrides the datatype of x_data 覆盖 x_data 的数据类型
print(f"Random Tensor: \n {x_rand} \n")
Out:
Ones Tensor:
tensor([[1, 1],
[1, 1]])
Random Tensor:
tensor([[0.3277, 0.7579],
[0.1860, 0.8509]])
定义tensor的大小形状(shape),并用常量值或随机值填充
shape = (2,3,)
rand_tensor = torch.rand(shape)
ones_tensor = torch.ones(shape)
zeros_tensor = torch.zeros(shape)
print(f"Random Tensor: \n {rand_tensor} \n")
print(f"Ones Tensor: \n {ones_tensor} \n")
print(f"Zeros Tensor: \n {zeros_tensor}")
Out:
Random Tensor:
tensor([[0.2882, 0.0322, 0.4411],
[0.5961, 0.6428, 0.1681]])
Ones Tensor:
tensor([[1., 1., 1.],
[1., 1., 1.]])
Zeros Tensor:
tensor([[0., 0., 0.],
[0., 0., 0.]])
tensor可用的一些属性或者说接口
shape dtype device
tensor = torch.rand(3,4)
print(f"Shape of tensor: {tensor.shape}")
print(f"Datatype of tensor: {tensor.dtype}")
print(f"Device tensor is stored on: {tensor.device}")
Out:
Shape of tensor: torch.Size([3, 4])
Datatype of tensor: torch.float32
Device tensor is stored on: cpu
tensor的运算操作
和python或者说matlab的语法差不多 很容易理解
tensor = torch.ones(4, 4)
print('First row: ', tensor[0])
print('First column: ', tensor[:, 0])
print('Last column:', tensor[..., -1])
tensor[:,1] = 0
print(tensor)
Out:
First row: tensor([1., 1., 1., 1.])
First column: tensor([1., 1., 1., 1.])
Last column: tensor([1., 1., 1., 1.])
tensor([[1., 0., 1., 1.],
[1., 0., 1., 1.],
[1., 0., 1., 1.],
[1., 0., 1., 1.]])
连接操作
t1 = torch.cat([tensor, tensor, tensor], dim=1)
print(t1)
Out:
tensor([[1., 0., 1., 1., 1., 0., 1., 1., 1., 0., 1., 1.],
[1., 0., 1., 1., 1., 0., 1., 1., 1., 0., 1., 1.],
[1., 0., 1., 1., 1., 0., 1., 1., 1., 0., 1., 1.],
[1., 0., 1., 1., 1., 0., 1., 1., 1., 0., 1., 1.]])
算数运算
# This computes the matrix multiplication between two tensors. y1, y2, y3 will have the same value #tensor矩阵乘法,y1,y2,y3都是矩阵乘法结果 y1 = tensor @ tensor.T y2 = tensor.matmul(tensor.T) y3 = torch.rand_like(tensor) torch.matmul(tensor, tensor.T, out=y3) # This computes the element-wise product. z1, z2, z3 will have the same value # tensor点乘 tensor逐个元素相互乘积 z1 = tensor * tensor z2 = tensor.mul(tensor) z3 = torch.rand_like(tensor) torch.mul(tensor, tensor, out=z3)
单元素张量:如果您有一个单元素张量,例如通过将张量的所有值聚合为一个值,您可以使用 item() 将其转换为 Python 数值
agg = tensor.sum()
agg_item = agg.item()
print(agg_item, type(agg_item))
Out:
12.0 <class 'float'>
就地操作 将结果存储到操作数中的操作称为就地操作。 它们由“_”后缀表示。 例如:: x.copy_(y)
, x.t_()
, will change x
.
print(tensor, "\n")
tensor.add_(5)
print(tensor)
Out:
tensor([[1., 0., 1., 1.],
[1., 0., 1., 1.],
[1., 0., 1., 1.],
[1., 0., 1., 1.]])
tensor([[6., 5., 6., 6.],
[6., 5., 6., 6.],
[6., 5., 6., 6.],
[6., 5., 6., 6.]])
处理数据样本的代码可能会变得混乱且难以维护; 我们理想地希望我们的数据集代码与我们的模型训练代码分离,以获得更好的可读性和模块化。 PyTorch 提供了两个数据原语:torch.utils.data.DataLoader 和 torch.utils.data.Dataset,它们允许您使用预加载的数据集以及您自己的数据。 Dataset 存储样本及其对应的标签,DataLoader 在 Dataset 周围包装一个可迭代对象,以便轻松访问样本。
PyTorch 域库提供了许多预加载的数据集(例如 FashionMNIST),它们是 torch.utils.data.Dataset 的子类并实现特定于特定数据的功能。 它们可用于对您的模型进行原型设计和基准测试。
Load Dataset的相关参数 以FashionMNIST Dataset为例
root
is the path where the train/test data is stored, 简单来说 地址,数据集放置或者要下载的位置
train
specifies training or test dataset,训练集还是测试集
download=True
downloads the data from the internet if it’s not available at root
. 是否从pytorch官方下载数据集
transform
and target_transform
specify the feature and label transformations指定特征和标签转换 指定使用怎样的transform
import torch from torch.utils.data import Dataset from torchvision import datasets from torchvision.transforms import ToTensor import matplotlib.pyplot as plt training_data = datasets.FashionMNIST( root="data", train=True, download=True, transform=ToTensor() ) test_data = datasets.FashionMNIST( root="data", train=False, download=True, transform=ToTensor() )
可视化数据集
我们可以像列表一样手动索引数据集:training_data[index]。 我们使用 matplotlib 来可视化训练数据中的一些样本。
labels_map = { 0: "T-Shirt", 1: "Trouser", 2: "Pullover", 3: "Dress", 4: "Coat", 5: "Sandal", 6: "Shirt", 7: "Sneaker", 8: "Bag", 9: "Ankle Boot", } figure = plt.figure(figsize=(8, 8)) cols, rows = 3, 3 for i in range(1, cols * rows + 1): sample_idx = torch.randint(len(training_data), size=(1,)).item() img, label = training_data[sample_idx] figure.add_subplot(rows, cols, i) plt.title(labels_map[label]) plt.axis("off") plt.imshow(img.squeeze(), cmap="gray") plt.show()
创建自定义的数据集
自定义的数据集必须包含:
: __init__, __len__, 和 __getitem__.
三个函数
例如 以FashionMNIST数据集为例
图像存储在目录 img_dir 中,它们的标签单独存储在 CSV 文件 annotations_file 中。
import os import pandas as pd from torchvision.io import read_image class CustomImageDataset(Dataset): def __init__(self, annotations_file, img_dir, transform=None, target_transform=None): self.img_labels = pd.read_csv(annotations_file) self.img_dir = img_dir self.transform = transform self.target_transform = target_transform def __len__(self): return len(self.img_labels) def __getitem__(self, idx): img_path = os.path.join(self.img_dir, self.img_labels.iloc[idx, 0]) image = read_image(img_path) label = self.img_labels.iloc[idx, 1] if self.transform: image = self.transform(image) if self.target_transform: label = self.target_transform(label) return image, label
__init__
函数
__init__
函数在实例化 Dataset 对象时运行一次。 我们初始化包含图像、注释文件和两个转换的目录
labels.csv文件例如这样
tshirt1.jpg, 0
tshirt2.jpg, 0
......
ankleboot999.jpg, 9
def __init__(self, annotations_file, img_dir, transform=None, target_transform=None):
self.img_labels = pd.read_csv(annotations_file, names=['file_name', 'label'])
self.img_dir = img_dir
self.transform = transform
self.target_transform = target_transform
__len__
函数返回我们数据集中的样本数。
def __len__(self):
return len(self.img_labels)
__getitem__
函数从给定索引 idx 的数据集中加载并返回一个样本。 基于索引,它识别图像在磁盘上的位置,使用 read_image 将其转换为张量,从 self.img_labels 中的 csv 数据中检索相应的标签,调用它们的转换函数(如果适用),并返回张量图像 和元组中的相应标签。
def __getitem__(self, idx):
img_path = os.path.join(self.img_dir, self.img_labels.iloc[idx, 0])
image = read_image(img_path)
label = self.img_labels.iloc[idx, 1]
if self.transform:
image = self.transform(image)
if self.target_transform:
label = self.target_transform(label)
return image, label
利用数据集传递数据进行训练
数据集检索我们数据集的特征并一次标记一个样本。 在训练模型时,我们通常希望以“小批量”的形式传递样本,在每个时期重新洗牌数据以减少模型过度拟合,并使用 Python 的多处理来加速数据检索。
DataLoader 是一个迭代器,它在一个简单的 API 中为我们抽象了这种复杂性。
from torch.utils.data import DataLoader
train_dataloader = DataLoader(training_data, batch_size=64, shuffle=True)
test_dataloader = DataLoader(test_data, batch_size=64, shuffle=True)
遍历 DataLoader
我们已经将该数据集加载到 DataLoader 中,并且可以根据需要遍历数据集。 下面的每次迭代都会返回一批 train_features 和 train_labels(分别包含 batch_size=64 个特征和标签)。 因为我们指定了 shuffle=True,所以在我们遍历所有批次后,数据会被打乱
# Display image and label.
train_features, train_labels = next(iter(train_dataloader))
print(f"Feature batch shape: {train_features.size()}")
print(f"Labels batch shape: {train_labels.size()}")
img = train_features[0].squeeze()
label = train_labels[0]
plt.imshow(img, cmap="gray")
plt.show()
print(f"Label: {label}")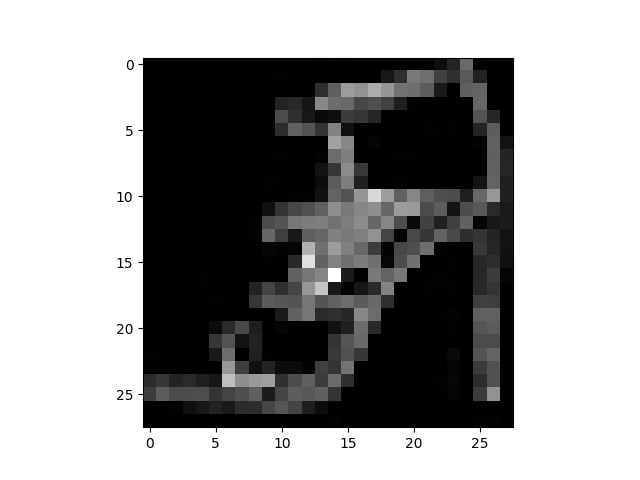
Out:
Feature batch shape: torch.Size([64, 1, 28, 28])
Labels batch shape: torch.Size([64])
Label: 5
简单来说,transform就是对data进行操作,使他成为适合我们网络操作的数据,即数据并不总是以训练机器学习算法所需的最终处理形式出现。 我们使用转换来对数据进行一些操作并使其适合训练。
所有 TorchVision 数据集都有两个参数——transform 用于修改特征,target_transform 用于修改标签——它们接受包含转换逻辑的可调用对象。 torchvision.transforms 模块提供了几种开箱即用的常用转换。
即幸运的是,pytorch集成了很好的transform函数,我们不用再费脑子去写新函数(应该)
import torch
from torchvision import datasets
from torchvision.transforms import ToTensor, Lambda
ds = datasets.FashionMNIST(
root="data",
train=True,
download=True,
transform=ToTensor(),
target_transform=Lambda(lambda y: torch.zeros(10, dtype=torch.float).scatter_(0, torch.tensor(y), value=1))
)
ToTensor()
ToTensor() 将 PIL 图像或 NumPy ndarray 转换为 FloatTensor。 并在 [0., 1.] 范围内缩放图像的像素强度值
神经网络由对数据执行操作的层/模块组成。 torch.nn 命名空间提供了构建自己的神经网络所需的所有构建块。 PyTorch 中的每个模块都是 nn.Module 的子类。 神经网络是一个模块本身,由其他模块(层)组成。 这种嵌套结构允许轻松构建和管理复杂的架构。
import os
import torch
from torch import nn
from torch.utils.data import DataLoader
from torchvision import datasets, transforms
选择使用的设备 cpu或gpu
device = 'cuda' if torch.cuda.is_available() else 'cpu'
print(f'Using {device} device')
定义类
我们通过继承 nn.Module 来定义我们的神经网络,并在 init 中初始化神经网络层。 每个 nn.Module 子类都在 forward 方法中实现对输入数据的操作。
class NeuralNetwork(nn.Module): def __init__(self): super(NeuralNetwork, self).__init__() self.flatten = nn.Flatten() self.linear_relu_stack = nn.Sequential( nn.Linear(28*28, 512), nn.ReLU(), nn.Linear(512, 512), nn.ReLU(), nn.Linear(512, 10), ) def forward(self, x): x = self.flatten(x) logits = self.linear_relu_stack(x) return logits
model = NeuralNetwork().to(device)
print(model)
网络结构 线性层+Relu层+线性层+relu层再到线性层
Out:
NeuralNetwork(
(flatten): Flatten(start_dim=1, end_dim=-1)
(linear_relu_stack): Sequential(
(0): Linear(in_features=784, out_features=512, bias=True)
(1): ReLU()
(2): Linear(in_features=512, out_features=512, bias=True)
(3): ReLU()
(4): Linear(in_features=512, out_features=10, bias=True)
)
)
为了使用模型,我们将输入数据传递给它。 这将执行模型的转发,以及一些后台操作。 不要直接调用model.forward()!
在输入上调用模型会返回一个 10 维张量,其中包含每个类的原始预测值。 我们通过将其传递给 nn.Softmax 模块的实例来获得预测概率。
X = torch.rand(1, 28, 28, device=device)
logits = model(X)
pred_probab = nn.Softmax(dim=1)(logits)
y_pred = pred_probab.argmax(1)
print(f"Predicted class: {y_pred}")
各个模块的作用,可以结合前一篇写的3Blue1Brown MLP原理来看
取一个由 3 张大小为 28x28 的图像组成的小批量样本,看看当我们通过网络传递它时会发生什么。
input_image = torch.rand(3,28,28)
print(input_image.size())
Out:
torch.Size([3, 28, 28])
我们初始化 [nn.Flatten]层以将每个 2D 28x28 图像转换为 784 个像素值的连续数组(小批量维度 (在dim=0 时)被保持)。
flatten = nn.Flatten()
flat_image = flatten(input_image)
print(flat_image.size())
Out:
torch.Size([3, 784])
nn.Linear是一个模块,它使用其存储的权重和偏差对输入应用线性变换。
layer1 = nn.Linear(in_features=28*28, out_features=20)
hidden1 = layer1(flat_image)
print(hidden1.size())
Out:
torch.Size([3, 20])
nn.ReLU非线性激活是在模型的输入和输出之间创建复杂映射的原因。 它们在线性变换之后被应用以引入非线性,帮助神经网络学习各种各样的现象。
在这个模型中,我们在我们的线性层之间使用 [nn.ReLU],但是还有其他的激活来在你的模型中引入非线性。
print(f"Before ReLU: {hidden1}\n\n")
hidden1 = nn.ReLU()(hidden1)
print(f"After ReLU: {hidden1}")
Out:
Before ReLU: tensor([[-0.5059, 0.0748, -0.3764, 0.2702, -0.5308, 0.1879, -0.1396, -0.1809, -0.0651, 0.1935, 0.8745, 0.3594, -0.0366, 0.4182, -0.4431, 0.2117, -0.2114, -0.0045, -0.2030, -0.5195], [-0.6142, -0.0293, 0.1397, 0.2526, -0.2365, 0.2625, -0.2154, -0.1611, -0.0842, -0.0181, 0.8274, 0.0739, 0.2244, 0.3389, -0.2915, 0.0280, 0.0083, -0.3871, -0.3059, -0.0009], [-0.4356, 0.0336, 0.0208, 0.5318, -0.4322, 0.2168, 0.1233, -0.2511, -0.0217, -0.0147, 0.6722, 0.0350, 0.0696, 0.6650, -0.3241, 0.0591, -0.1873, 0.0044, -0.1151, -0.3288]], grad_fn=<AddmmBackward0>) After ReLU: tensor([[0.0000, 0.0748, 0.0000, 0.2702, 0.0000, 0.1879, 0.0000, 0.0000, 0.0000, 0.1935, 0.8745, 0.3594, 0.0000, 0.4182, 0.0000, 0.2117, 0.0000, 0.0000, 0.0000, 0.0000], [0.0000, 0.0000, 0.1397, 0.2526, 0.0000, 0.2625, 0.0000, 0.0000, 0.0000, 0.0000, 0.8274, 0.0739, 0.2244, 0.3389, 0.0000, 0.0280, 0.0083, 0.0000, 0.0000, 0.0000], [0.0000, 0.0336, 0.0208, 0.5318, 0.0000, 0.2168, 0.1233, 0.0000, 0.0000, 0.0000, 0.6722, 0.0350, 0.0696, 0.6650, 0.0000, 0.0591, 0.0000, 0.0044, 0.0000, 0.0000]], grad_fn=<ReluBackward0>)
[nn.Sequential] 是一个有序的模块容器。 数据按照定义的相同顺序通过所有模块。 您可以使用顺序容器将快速网络组合在一起,如“seq_modules”。
seq_modules = nn.Sequential(
flatten,
layer1,
nn.ReLU(),
nn.Linear(20, 10)
)
input_image = torch.rand(3,28,28)
logits = seq_modules(input_image)
神经网络的最后一个线性层返回 logits - [-infty, infty] 中的原始值 - 传递给 [nn.Softmax] 模块。 logits 被缩放到值 [0, 1],代表模型对每个类别的预测概率。 dim
参数指示值必须总和为 1 的维度。
softmax = nn.Softmax(dim=1)
pred_probab = softmax(logits)
神经网络内的许多层都是参数化的,即具有在训练期间优化的相关权重和偏差。 子类 nn.Module 会自动跟踪模型对象中定义的所有字段,并使用模型的 parameters() 或 named_parameters() 方法使所有参数都可以访问。
我们迭代每个参数,并打印它的大小和它的值
print("Model structure: ", model, "\n\n")
for name, param in model.named_parameters():
print(f"Layer: {name} | Size: {param.size()} | Values : {param[:2]} \n")
Out:
Model structure: NeuralNetwork( (flatten): Flatten(start_dim=1, end_dim=-1) (linear_relu_stack): Sequential( (0): Linear(in_features=784, out_features=512, bias=True) (1): ReLU() (2): Linear(in_features=512, out_features=512, bias=True) (3): ReLU() (4): Linear(in_features=512, out_features=10, bias=True) ) ) Layer: linear_relu_stack.0.weight | Size: torch.Size([512, 784]) | Values : tensor([[-0.0088, 0.0077, 0.0342, ..., -0.0352, -0.0216, 0.0057], [ 0.0218, 0.0252, -0.0121, ..., -0.0119, -0.0242, -0.0097]], device='cuda:0', grad_fn=<SliceBackward0>) Layer: linear_relu_stack.0.bias | Size: torch.Size([512]) | Values : tensor([-0.0026, -0.0315], device='cuda:0', grad_fn=<SliceBackward0>) Layer: linear_relu_stack.2.weight | Size: torch.Size([512, 512]) | Values : tensor([[-0.0361, -0.0067, -0.0310, ..., 0.0283, 0.0308, 0.0301], [ 0.0113, -0.0117, 0.0122, ..., -0.0277, 0.0144, -0.0243]], device='cuda:0', grad_fn=<SliceBackward0>) Layer: linear_relu_stack.2.bias | Size: torch.Size([512]) | Values : tensor([-0.0320, 0.0118], device='cuda:0', grad_fn=<SliceBackward0>) Layer: linear_relu_stack.4.weight | Size: torch.Size([10, 512]) | Values : tensor([[ 0.0066, -0.0132, -0.0337, ..., 0.0186, -0.0261, -0.0128], [ 0.0329, 0.0164, 0.0112, ..., 0.0183, -0.0094, 0.0095]], device='cuda:0', grad_fn=<SliceBackward0>) Layer: linear_relu_stack.4.bias | Size: torch.Size([10]) | Values : tensor([0.0200, 0.0429], device='cuda:0', grad_fn=<SliceBackward0>)
在训练神经网络时,最常用的算法是反向传播。 在该算法中,参数(模型权重)根据损失函数相对于给定参数的梯度进行调整。
为了计算这些梯度,PyTorch 有一个名为 torch.autograd 的内置微分引擎。 它支持任何计算图的梯度自动计算。
考虑最简单的一层神经网络,输入 x,参数 w 和 b,以及一些损失函数。 它可以通过以下方式在 PyTorch 中定义:
import torch
x = torch.ones(5) # input tensor
y = torch.zeros(3) # expected output
w = torch.randn(5, 3, requires_grad=True)
b = torch.randn(3, requires_grad=True)
z = torch.matmul(x, w)+b
loss = torch.nn.functional.binary_cross_entropy_with_logits(z, y)
仔细看代码 即求出交叉熵
张量、函数和计算图等概念
This code defines the following computational graph:
简单来说,如果你学过信号与系统或者自动控制原理,就和里面那个信号流图非常相似
在这个网络中,w 和 b 是我们需要优化的参数。 因此,我们需要能够计算关于这些变量的损失函数的梯度。 为了做到这一点,我们设置了这些张量的 requires_grad 属性。
我们应用于张量来构建计算图的函数实际上是类 Function 的对象。 该对象知道如何在前向计算函数,以及如何在反向传播步骤中计算其导数。 对反向传播函数的引用存储在张量的 grad_fn 属性中。
print('Gradient function for z =', z.grad_fn)
print('Gradient function for loss =', loss.grad_fn)
Out:
Gradient function for z = <AddBackward0 object at 0x7f1127a750b8>
Gradient function for loss = <BinaryCrossEntropyWithLogitsBackward0 object at 0x7f1127a750b8>
计算导数
为了优化神经网络中参数的权重,我们需要计算损失函数对参数的导数,即我们需要
∂
l
o
s
s
∂
w
\frac{\partial loss}{\partial w}
∂w∂loss
和
∂
l
o
s
s
∂
b
\frac{\partial loss}{\partial b}
∂b∂loss
在一些固定的 x 和 y 值下。 为了计算这些导数,我们调用 loss.backward(),然后利用 w.grad 和 b.grad可以查看求出的值
loss.backward()
print(w.grad)
print(b.grad)
Out:
tensor([[0.0093, 0.0089, 0.1828],
[0.0093, 0.0089, 0.1828],
[0.0093, 0.0089, 0.1828],
[0.0093, 0.0089, 0.1828],
[0.0093, 0.0089, 0.1828]])
tensor([0.0093, 0.0089, 0.1828])
注意
我们只能获取计算图的叶子节点的 grad 属性,这些节点的 requires_grad 属性设置为 True。 对于我们图中的所有其他节点,渐变将不可用。
出于性能原因,我们只能在给定的图形上使用“向后”一次执行梯度计算。 如果我们需要在同一个图上进行多次 backward
调用,我们需要将 retain_graph=True
传递给 backward
调用。
一些细节
从概念上讲,autograd 在一个由 [Function] 组成的有向无环图 (DAG) 中保存数据(张量)和所有已执行操作(以及由此产生的新张量)的记录 对象。 在这个 DAG 中,叶子是输入张量,根是输出张量。 通过从根到叶跟踪此图,您可以使用链式法则自动计算梯度
在前向传递中,autograd 同时做两件事:
当在 DAG 根上调用 .backward()
时,反向传递开始。 autograd
然后:
计算每个.grad_fn
的梯度,
.grad
属性中累加它们 -使用链式法则,一直传播到叶张量。
现在我们有了模型和数据,是时候通过优化数据参数来训练、验证和测试我们的模型了。 训练模型是一个迭代过程; 在每次迭代(称为 epoch)中,模型对输出进行猜测,计算其猜测中的误差(损失),收集误差对其参数的导数(如我们在上一节中看到的),并优化 这些参数使用梯度下降。
Copyright © 2003-2013 www.wpsshop.cn 版权所有,并保留所有权利。