赞
踩
决策树(Decision Tree)分类技术是一种比较直观的用来分析不确定性事件的概率模型,属于数据挖掘技术中比较常见的一种方法。主要是用在分析和评价项目预期的风险和可行性的问题。决策树作为预测模型,从直观可以看作类似于一棵树,从树根到各个分支都可以看作一个如何分类的问题。枝干上的每一片树叶代表了具有分类功能的样本数据的分割。本次的实验将构建决策树模型来对客户是否违约进行预测分析。
此次实验的步骤如下:
1、导入实验相关的库:
#基础数据准备 import pandas as pd import numpy as np ##可视化 import matplotlib.pyplot as plt %matplotlib inline import seaborn as sns from pylab import mpl mpl.rcParams['font.sans-serif'] = ['SimHei'] mpl.rcParams['axes.unicode_minus'] =False ## 根据卡方检验选取关联特征 from sklearn.feature_selection import SelectKBest from sklearn.feature_selection import chi2 from sklearn.metrics import * # 导入决策树分类模型 from sklearn.tree import DecisionTreeClassifier
2、数据导入
data = pd.read_excel(r'客户信息及违约表现.xlsx')
展示数据的前五条数据
data.head(5)
output:
3、数据导入之后查看数据的情况(看是否是有缺失值,异常值)
data.describe()
data.isnull().sum()## 数据集没有缺失值
可以发现数据是没有缺失值的
4、特征工程:对收入数据和历史授信额度进行处理以及编码
首先对收入那一列的数据进行整理编码
data["收入"].describe()
从上面的数据查看中我们可以发现收入最小是1.0*exp(5) ,最大是6.602542exp(6),我的想法是可以将其分成10个收入范围,每个范围分别用0,1,2,3,。。。。9来表示(也可以分为更多的类)
income_max = max(data["收入"]) income_min = min(data["收入"]) a = round((income_max-income_min)*0.1,2) #定义一个给收入编码的函数getcode(x) def getcode(x): if x>=income_min and x<income_min+a*1: return 0 if x>=income_min+a*1 and x<income_min+a*2: return 1 if x>=income_min+a*2 and x<income_min+a*3: return 2 if x>=income_min+a*3 and x<income_min+a*4: return 3 if x>=income_min+a*4 and x<income_min+a*5: return 4 if x>=income_min+a*5 and x<income_min+a*6: return 5 if x>=income_min+a*6 and x<income_min+a*7: return 6 if x>=income_min+a*7 and x<income_min+a*8: return 7 if x>=income_min+a*8 and x<income_min+a*9: return 8 if x>=income_min+a*9 and x<=income_max: return 9 ##对收入进行编码 data["income"]=data["收入"].apply(lambda x:getcode(x)) #查看收入的不同编码的个数并做数据可视化(直方图展示) data["income"].value_counts() data["income"].hist(bins=20)
plt.axis("equal")#保证绘制的图形是一个圆,而不是一个椭圆
plt.title("income收入的数据分布",fontsize=18,color="#000033")
plt.pie(data["income"].value_counts(),autopct="%.2f%%",)
plt.legend(data["income"].unique())
``
`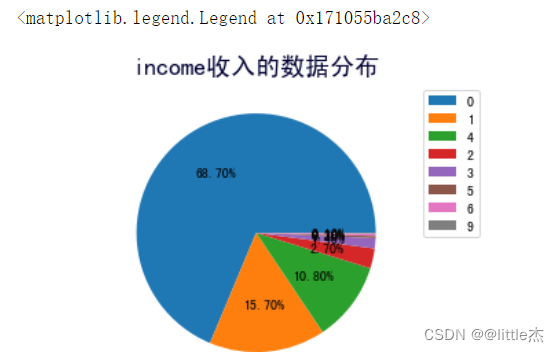
对客户的收入信息预处理之后,对历史授信额度的数据进行编码
```python
data["历史授信额度"].describe()
从上面可以发现最小是0 ,说明用户没有授权历史额度,将这种情况单独归为一类,可以将剩下的分成10个收入范围,每个范围分别用1,2,3,。。。。10来表示
credit_max = max(data["历史授信额度"]) credit_min = min(data["历史授信额度"]) credit_one = round((credit_max-credit_min)*0.1,2)##保留两位小数 print(credit_one) #定义一个给历史授信额度编码的函数getCreditCode(x) def getCreditCode(x): if x==0: return 0 if x>credit_min and x<=credit_min+credit_one*1: return 1 if x>credit_min+credit_one*1 and x<=credit_min+credit_one*2: return 2 if x>credit_min+credit_one*2 and x<=credit_min+credit_one*3: return 3 if x>credit_min+credit_one*3 and x<=credit_min+credit_one*4: return 4 if x>credit_min+credit_one*4 and x<=credit_min+credit_one*5: return 5 if x>credit_min+credit_one*5 and x<=credit_min+credit_one*6: return 6 if x>credit_min+credit_one*6 and x<=credit_min+credit_one*7: return 7 if x>credit_min+credit_one*7 and x<=credit_min+credit_one*8: return 8 if x>credit_min+credit_one*8 and x<=credit_min+credit_one*9: return 9 if x>credit_min+credit_one*9 and x<=credit_max: return 10 ##对收入进行编码 data["credit"]=data["历史授信额度"].apply(lambda x:getCreditCode(x)) ## 查看额度编码后的数据量(果然实际上的类别小于等于11,只有7个类别) data["credit"].value_counts() data["credit"].hist(bins=20)
plt.axis("equal")#保证绘制的图形是一个圆,而不是一个椭圆
plt.title("credit历史授信额度的数据分布",fontsize=18,color="#000033")
plt.pie(data["credit"].value_counts(),autopct="%.2f%%",)
plt.legend(data["credit"].unique())
最后,将数据中收入和历史授信额度这两列数据剔除
data.drop(columns=["收入","历史授信额度"],inplace=True)
数据可视化查看:
data.hist(bins=20,figsize=(10,10))
5、查看数据的分布是否均匀
data.groupby("是否违约").count()
可以发现为0的样本数量(未违约)占总样本的数量的3/5,为1的样本数量(违约)占总样本的数量的2/5, 说明两种特征的样本数量比较均衡,可以构建分类模型
6、关联分析
## 计算协方差
dataCorr = data.corr()
dataCorr
## 热力图展现相关关系
import seaborn as sns
plt.rcParams['font.sans-serif'] = ['SimHei']
plt.rcParams['axes.unicode_minus'] = False
plt.figure(figsize=(20,10))
sns.heatmap(dataCorr,annot=False,fmt="float",linewidths=0.5,cmap="RdYlBu")
plt.tick_params(labelsize=20)
font1={'family':'Times New Roman',
'weight':'normal',
'size':20,
}
plt.show
计算“是否违约”与其他特征变量的关系
dataCorr = data.corr()["是否违约"]
dataCorr
7、卡方检验
选择相关性较强的特征
## 1、卡方检验 dataFeature = data.drop(columns=['是否违约']) ## 根据卡方检验选取关联特征 from sklearn.feature_selection import SelectKBest from sklearn.feature_selection import chi2 #选择k个最佳特征 s = SelectKBest(chi2,k=4).fit(dataFeature,data['是否违约']) feat_scores=pd.DataFrame(index=[0,1,2,3,4]) feat_scores["F score"] = pd.DataFrame(s.scores_) feat_scores["P value"] = pd.DataFrame(s.pvalues_) feat_scores["是否相关"] = pd.DataFrame(s.get_support()) feat_scores
## 根据卡方检验选取关联特征 from sklearn.feature_selection import SelectKBest from sklearn.feature_selection import chi2 name = [] feature = [] for i in dataFeature.columns: name.append(i) X_new = SelectKBest(chi2,k=4).fit(dataFeature,data['是否违约']) outcome = X_new.get_support() for i in range(0,len(name)): if outcome[i]: feature.append(name[i]) result = pd.DataFrame({"特征":name, "F score":feat_scores["F score"], "P value":feat_scores["P value"], "是否相关":outcome}) result
选取的预测特征为feature:[‘年龄’, ‘历史违约次数’, ‘income’, ‘credit’]
feature.append("是否违约")
dataFeatureSelect=data[feature]
dataFeatureSelect
8、模型的构建与评估
from sklearn.model_selection import train_test_split #1 进行训练集和测试集的拆分 x_train,x_test = train_test_split(dataFeatureSelect, train_size=0.8, test_size=0.2, shuffle=True) ## 2、决策树模型构建 ### 决策树模型既可以用作分类,又可以用作回归 # 导入相关的包 from sklearn.tree import DecisionTreeClassifier dtc = DecisionTreeClassifier().fit(x_train.drop( columns=['是否违约']), x_train['是否违约']) #绘制决策树图 from sklearn.tree import export_graphviz export_graphviz(dtc, out_file="tree.dot",filled=True,rounded=True) #以PDF格式输出决策树图 import graphviz with(open("tree.dot")) as f: dot_graph=f.read() dot=graphviz.Source(dot_graph) dot.view() ## 3、利用模型进行预测 predictedTreeData = dtc.predict(x_test.drop(columns=['是否违约'])) ## 4、对用户是否违约识别决策树模型进行评估 print("accuracy_score_tree:",accuracy_score(x_test['是否违约'],predictedTreeData)) print("precision_score_tree:",precision_score(x_test['是否违约'],predictedTreeData)) print("recall_score_tree:",recall_score(x_test['是否违约'],predictedTreeData))
## 5、对测试集评判模型预测效果
df_predict_tree = pd.DataFrame(x_test,columns=['是否违约'])
df_predict_tree['prediction_dtc'] =predictedTreeData
df_predict_tree.head(10)
Copyright © 2003-2013 www.wpsshop.cn 版权所有,并保留所有权利。